2023
Kamp, Michael; Fischer, Jonas; Vreeken, Jilles
Federated Learning from Small Datasets Proceedings Article
In: International Conference on Learning Representations (ICLR), 2023.
Links | BibTeX | Tags: black-box, black-box parallelization, daisy, daisy-chaining, FedDC, federated learning, small, small datasets
@inproceedings{kamp2023federated,
title = {Federated Learning from Small Datasets},
author = {Michael Kamp and Jonas Fischer and Jilles Vreeken},
url = {https://michaelkamp.org/wp-content/uploads/2022/08/FederatedLearingSmallDatasets.pdf},
year = {2023},
date = {2023-05-01},
urldate = {2023-05-01},
booktitle = {International Conference on Learning Representations (ICLR)},
journal = {arXiv preprint arXiv:2110.03469},
keywords = {black-box, black-box parallelization, daisy, daisy-chaining, FedDC, federated learning, small, small datasets},
pubstate = {published},
tppubtype = {inproceedings}
}
David Kaltenpoth Osman Mian, Michael Kamp
Nothing but Regrets - Privacy-Preserving Federated Causal Discovery Proceedings Article
In: International Conference on Artificial Intelligence and Statistics (AISTATS), 2023.
BibTeX | Tags: causal discovery, causality, explainable, federated, federated causal discovery, federated learning, interpretable
@inproceedings{mian2022nothing,
title = {Nothing but Regrets - Privacy-Preserving Federated Causal Discovery},
author = {Osman Mian, David Kaltenpoth, Michael Kamp, Jilles Vreeken},
year = {2023},
date = {2023-04-25},
urldate = {2023-04-25},
booktitle = {International Conference on Artificial Intelligence and Statistics (AISTATS)},
keywords = {causal discovery, causality, explainable, federated, federated causal discovery, federated learning, interpretable},
pubstate = {published},
tppubtype = {inproceedings}
}
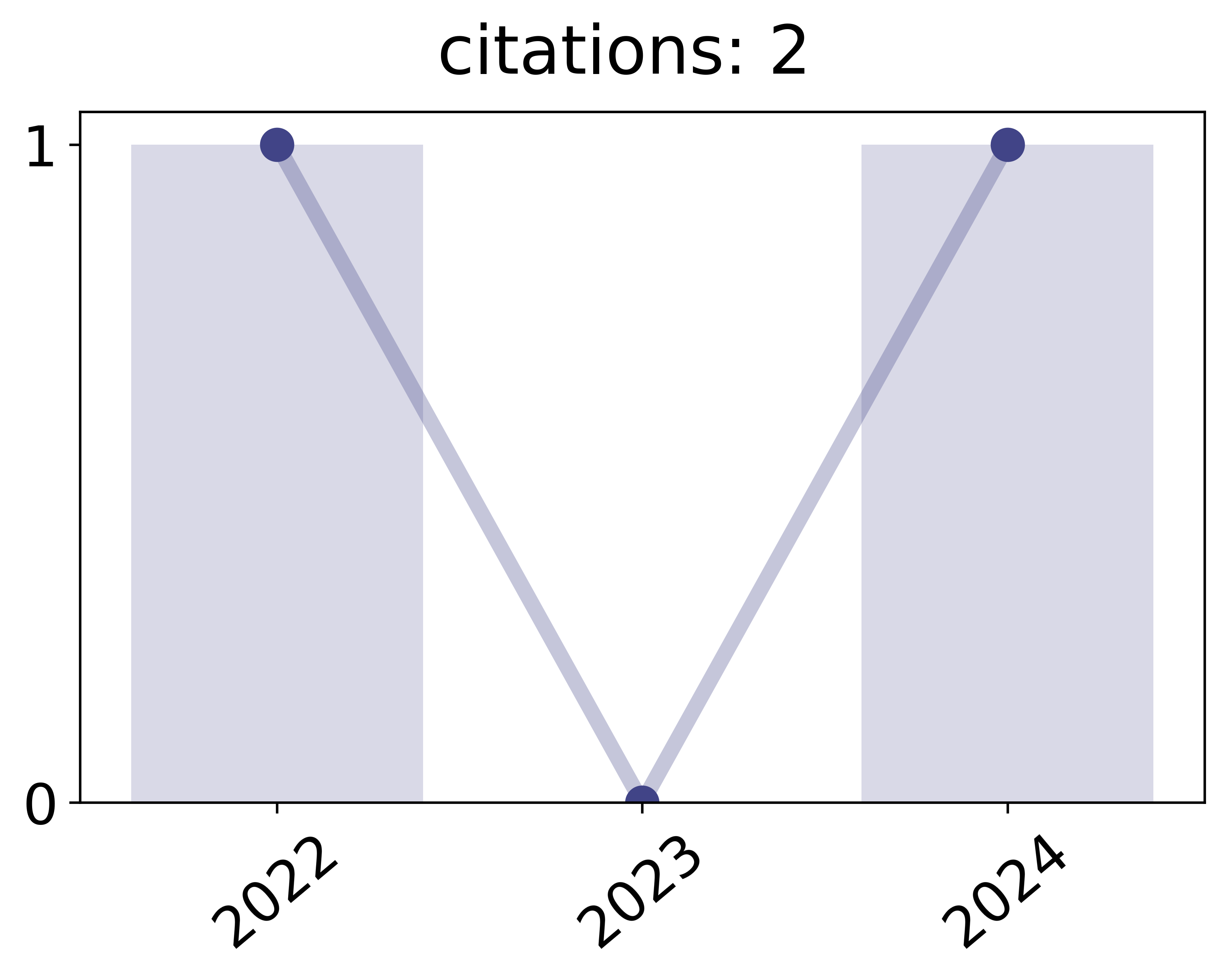
Mian, Osman; Kamp, Michael; Vreeken, Jilles
Information-Theoretic Causal Discovery and Intervention Detection over Multiple Environments Proceedings Article
In: Proceedings of the AAAI Conference on Artificial Intelligence (AAAI), 2023.
BibTeX | Tags: causal discovery, causality, federated, federated causal discovery, federated learning, intervention
@inproceedings{mian2023informationb,
title = {Information-Theoretic Causal Discovery and Intervention Detection over Multiple Environments},
author = {Osman Mian and Michael Kamp and Jilles Vreeken},
year = {2023},
date = {2023-02-07},
urldate = {2023-02-07},
booktitle = {Proceedings of the AAAI Conference on Artificial Intelligence (AAAI)},
keywords = {causal discovery, causality, federated, federated causal discovery, federated learning, intervention},
pubstate = {published},
tppubtype = {inproceedings}
}
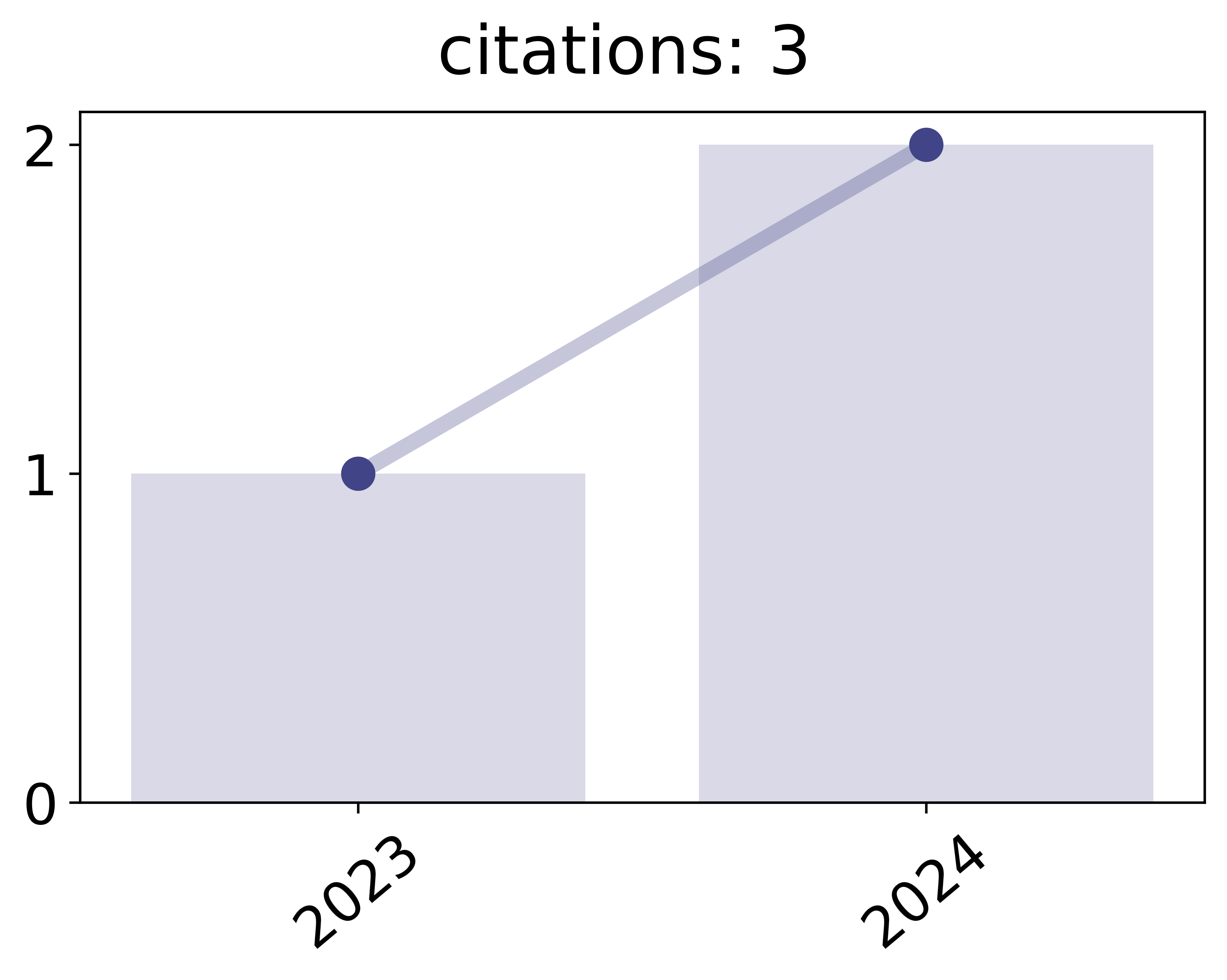
2021
Linsner, Florian; Adilova, Linara; Däubener, Sina; Kamp, Michael; Fischer, Asja
Approaches to Uncertainty Quantification in Federated Deep Learning Workshop
Machine Learning and Principles and Practice of Knowledge Discovery in Databases: International Workshops of ECML PKDD 2021, vol. 2, Springer, 2021.
Links | BibTeX | Tags: federated learning, uncertainty
@workshop{linsner2021uncertainty,
title = {Approaches to Uncertainty Quantification in Federated Deep Learning},
author = {Florian Linsner and Linara Adilova and Sina Däubener and Michael Kamp and Asja Fischer},
url = {https://michaelkamp.org/wp-content/uploads/2022/04/federatedUncertainty.pdf},
year = {2021},
date = {2021-09-17},
urldate = {2021-09-17},
booktitle = {Machine Learning and Principles and Practice of Knowledge Discovery in Databases: International Workshops of ECML PKDD 2021},
issuetitle = {Workshop on Parallel, Distributed, and Federated Learning},
volume = {2},
pages = {128-145},
publisher = {Springer},
keywords = {federated learning, uncertainty},
pubstate = {published},
tppubtype = {workshop}
}
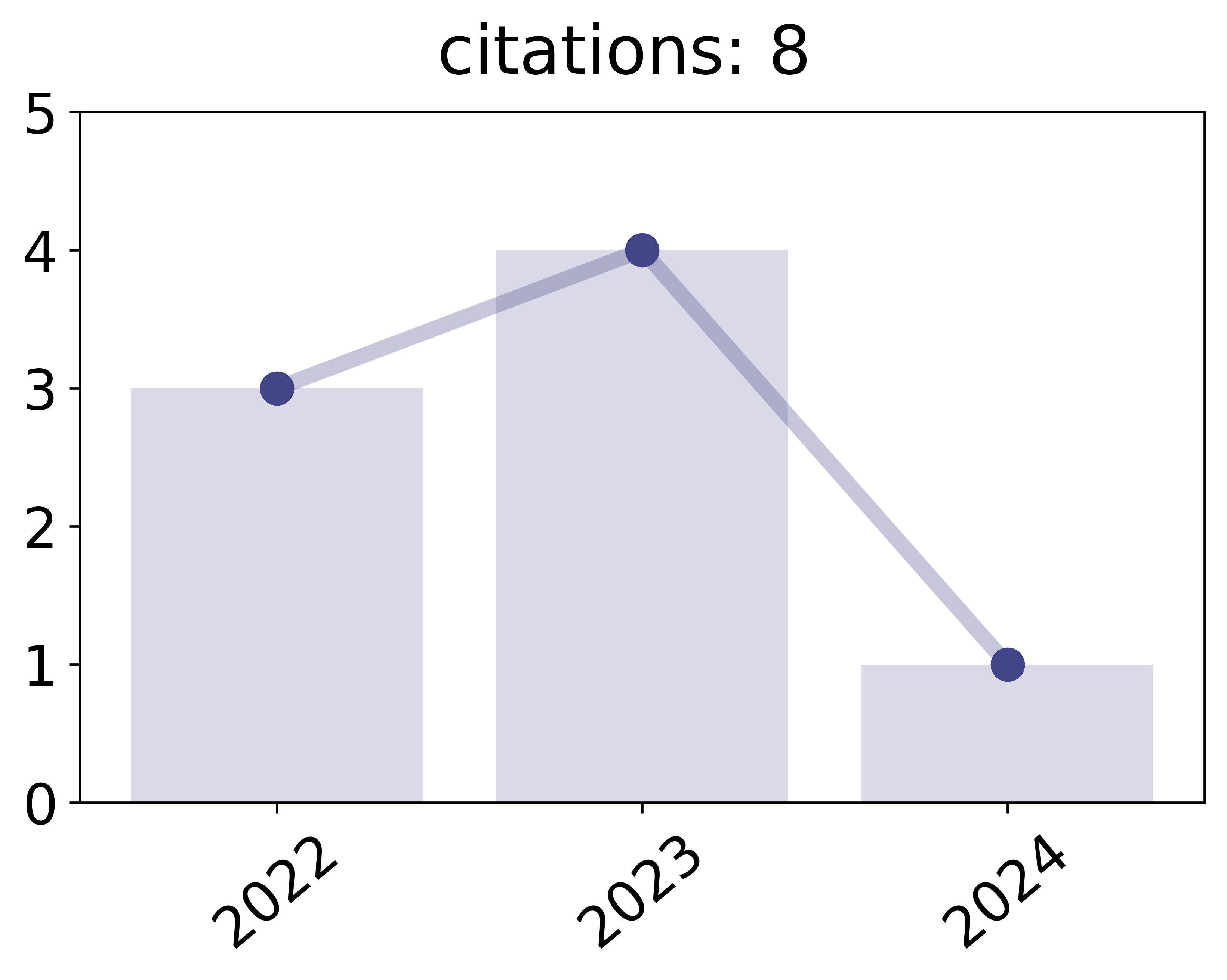
Li, Xiaoxiao; Jiang, Meirui; Zhang, Xiaofei; Kamp, Michael; Dou, Qi
FedBN: Federated Learning on Non-IID Features via Local Batch Normalization Proceedings Article
In: Proceedings of the 9th International Conference on Learning Representations (ICLR), 2021.
Abstract | Links | BibTeX | Tags: batch normalization, black-box parallelization, deep learning, federated learning
@inproceedings{li2021fedbn,
title = {FedBN: Federated Learning on Non-IID Features via Local Batch Normalization},
author = {Xiaoxiao Li and Meirui Jiang and Xiaofei Zhang and Michael Kamp and Qi Dou},
url = {https://michaelkamp.org/wp-content/uploads/2021/05/fedbn_federated_learning_on_non_iid_features_via_local_batch_normalization.pdf
https://michaelkamp.org/wp-content/uploads/2021/05/FedBN_appendix.pdf},
year = {2021},
date = {2021-05-03},
urldate = {2021-05-03},
booktitle = {Proceedings of the 9th International Conference on Learning Representations (ICLR)},
abstract = {The emerging paradigm of federated learning (FL) strives to enable collaborative training of deep models on the network edge without centrally aggregating raw data and hence improving data privacy. In most cases, the assumption of independent and identically distributed samples across local clients does not hold for federated learning setups. Under this setting, neural network training performance may vary significantly according to the data distribution and even hurt training convergence. Most of the previous work has focused on a difference in the distribution of labels or client shifts. Unlike those settings, we address an important problem of FL, e.g., different scanners/sensors in medical imaging, different scenery distribution in autonomous driving (highway vs. city), where local clients store examples with different distributions compared to other clients, which we denote as feature shift non-iid. In this work, we propose an effective method that uses local batch normalization to alleviate the feature shift before averaging models. The resulting scheme, called FedBN, outperforms both classical FedAvg, as well as the state-of-the-art for non-iid data (FedProx) on our extensive experiments. These empirical results are supported by a convergence analysis that shows in a simplified setting that FedBN has a faster convergence rate than FedAvg. Code is available at https://github.com/med-air/FedBN.},
keywords = {batch normalization, black-box parallelization, deep learning, federated learning},
pubstate = {published},
tppubtype = {inproceedings}
}
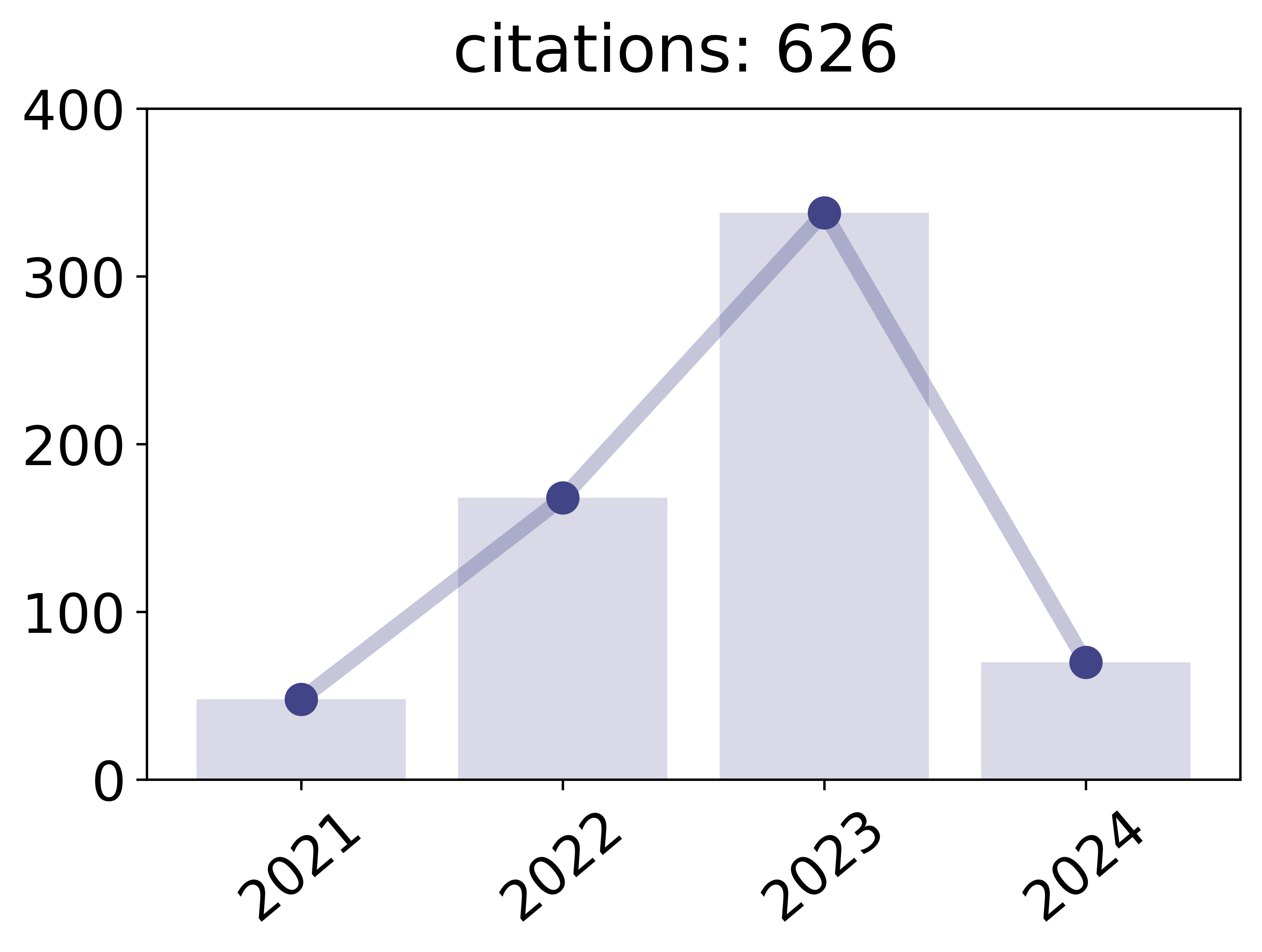
2018
Kamp, Michael; Adilova, Linara; Sicking, Joachim; Hüger, Fabian; Schlicht, Peter; Wirtz, Tim; Wrobel, Stefan
Efficient Decentralized Deep Learning by Dynamic Model Averaging Proceedings Article
In: Machine Learning and Knowledge Discovery in Databases, Springer, 2018.
Abstract | Links | BibTeX | Tags: decentralized, deep learning, federated learning
@inproceedings{kamp2018efficient,
title = {Efficient Decentralized Deep Learning by Dynamic Model Averaging},
author = {Michael Kamp and Linara Adilova and Joachim Sicking and Fabian Hüger and Peter Schlicht and Tim Wirtz and Stefan Wrobel},
url = {http://michaelkamp.org/wp-content/uploads/2018/07/commEffDeepLearning_extended.pdf},
year = {2018},
date = {2018-09-14},
urldate = {2018-09-14},
booktitle = {Machine Learning and Knowledge Discovery in Databases},
publisher = {Springer},
abstract = {We propose an efficient protocol for decentralized training of deep neural networks from distributed data sources. The proposed protocol allows to handle different phases of model training equally well and to quickly adapt to concept drifts. This leads to a reduction of communication by an order of magnitude compared to periodically communicating state-of-the-art approaches. Moreover, we derive a communication bound that scales well with the hardness of the serialized learning problem. The reduction in communication comes at almost no cost, as the predictive performance remains virtually unchanged. Indeed, the proposed protocol retains loss bounds of periodically averaging schemes. An extensive empirical evaluation validates major improvement of the trade-off between model performance and communication which could be beneficial for numerous decentralized learning applications, such as autonomous driving, or voice recognition and image classification on mobile phones.},
keywords = {decentralized, deep learning, federated learning},
pubstate = {published},
tppubtype = {inproceedings}
}
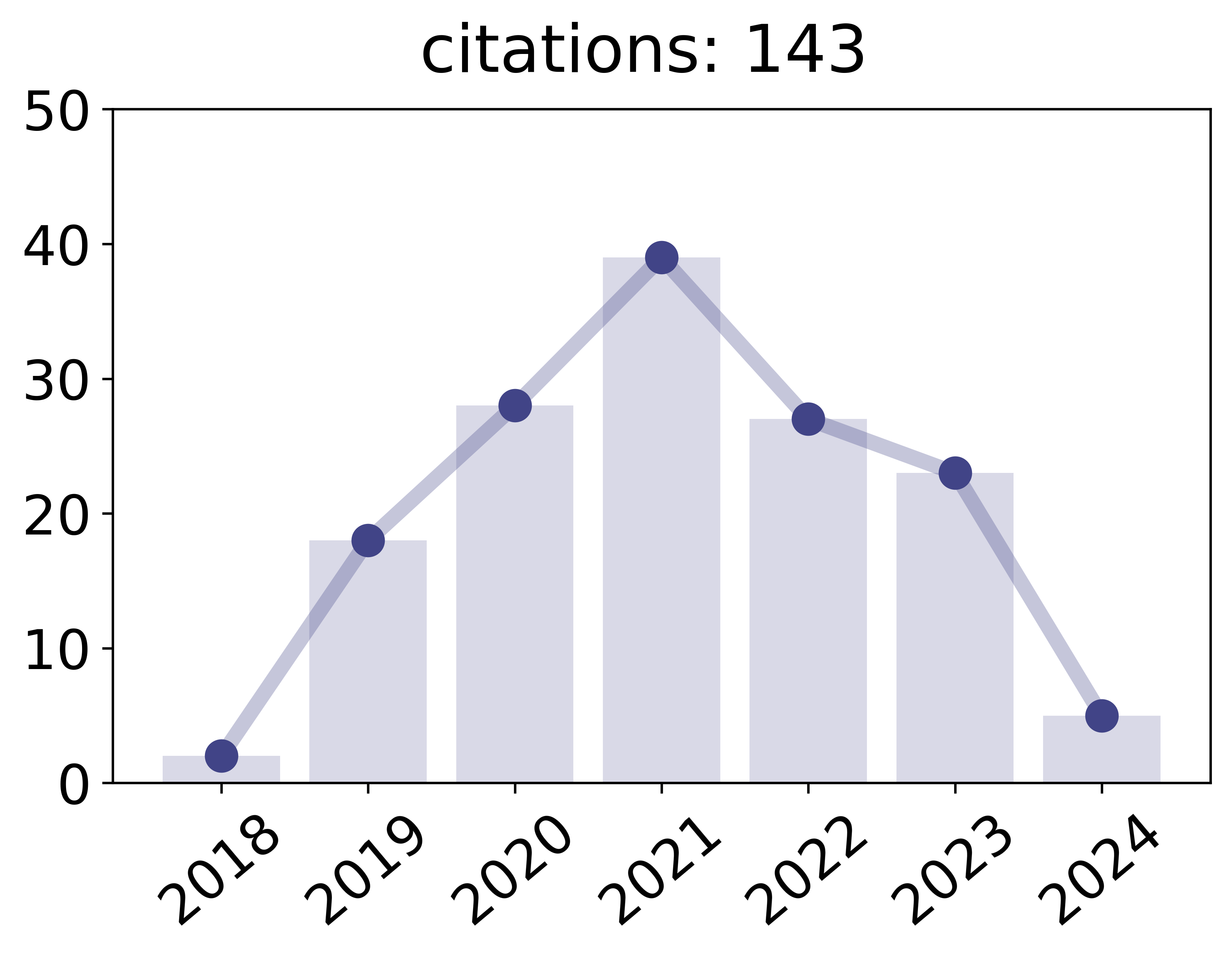
2016
Kamp, Michael; Bothe, Sebastian; Boley, Mario; Mock, Michael
Communication-Efficient Distributed Online Learning with Kernels Proceedings Article
In: Frasconi, Paolo; Landwehr, Niels; Manco, Giuseppe; Vreeken, Jilles (Ed.): Machine Learning and Knowledge Discovery in Databases, pp. 805–819, Springer International Publishing, 2016.
Abstract | Links | BibTeX | Tags: communication-efficient, distributed, dynamic averaging, federated learning, kernel methods, parallelization
@inproceedings{kamp2016communication,
title = {Communication-Efficient Distributed Online Learning with Kernels},
author = {Michael Kamp and Sebastian Bothe and Mario Boley and Michael Mock},
editor = {Paolo Frasconi and Niels Landwehr and Giuseppe Manco and Jilles Vreeken},
url = {http://michaelkamp.org/wp-content/uploads/2020/03/Paper467.pdf},
year = {2016},
date = {2016-09-16},
urldate = {2016-09-16},
booktitle = {Machine Learning and Knowledge Discovery in Databases},
pages = {805--819},
publisher = {Springer International Publishing},
abstract = {We propose an efficient distributed online learning protocol for low-latency real-time services. It extends a previously presented protocol to kernelized online learners that represent their models by a support vector expansion. While such learners often achieve higher predictive performance than their linear counterparts, communicating the support vector expansions becomes inefficient for large numbers of support vectors. The proposed extension allows for a larger class of online learning algorithms—including those alleviating the problem above through model compression. In addition, we characterize the quality of the proposed protocol by introducing a novel criterion that requires the communication to be bounded by the loss suffered.},
keywords = {communication-efficient, distributed, dynamic averaging, federated learning, kernel methods, parallelization},
pubstate = {published},
tppubtype = {inproceedings}
}
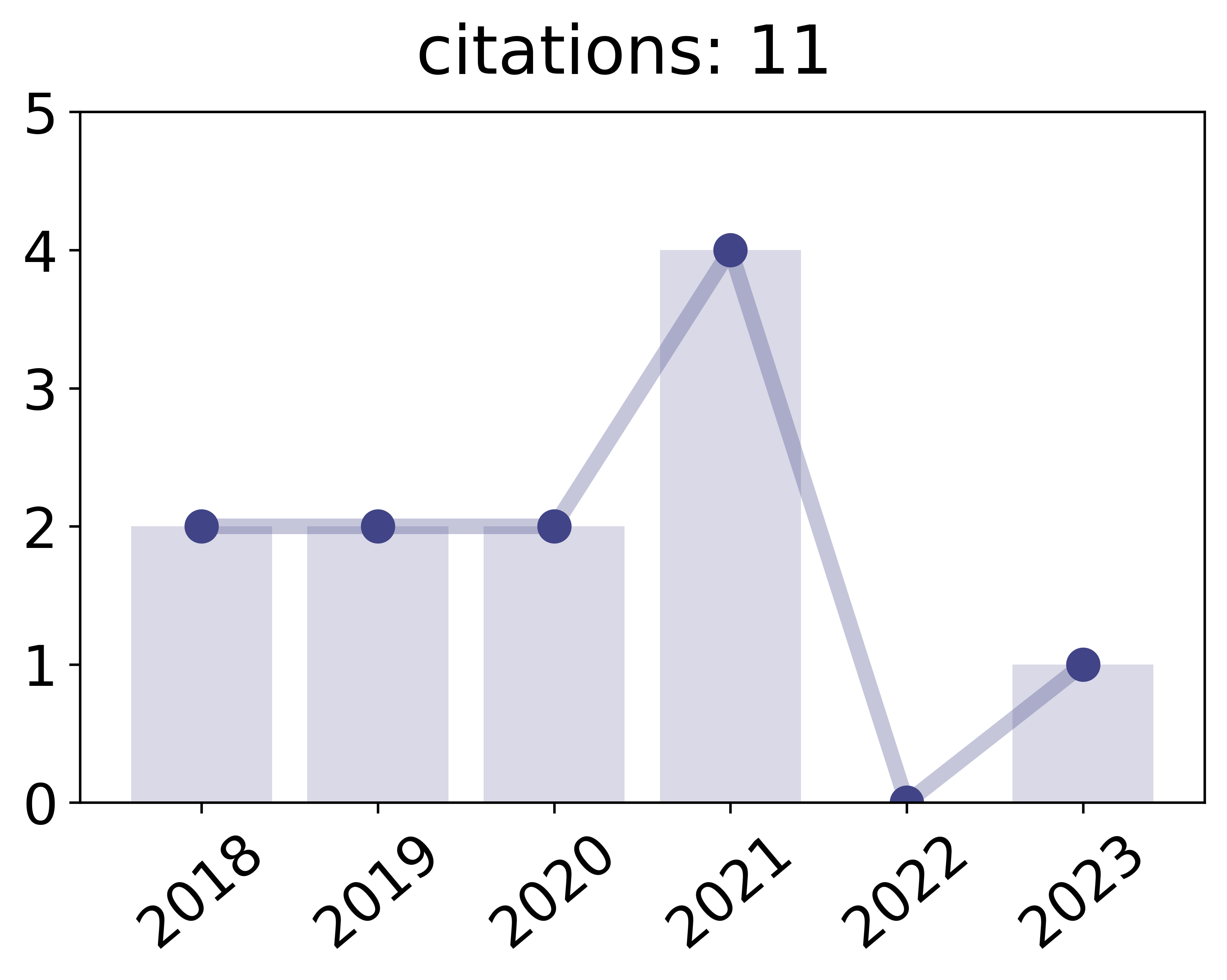