2024
Adilova, Linara; Andriushchenko, Maksym; Fischer, Michael Kamp Asja; Jaggi, Martin
Layer-wise Linear Mode Connectivity Proceedings Article
In: International Conference on Learning Representations (ICLR), Curran Associates, Inc, 2024.
Abstract | Links | BibTeX | Tags: deep learning, layer-wise, linear mode connectivity
@inproceedings{adilova2024layerwise,
title = {Layer-wise Linear Mode Connectivity},
author = {Linara Adilova and Maksym Andriushchenko and Michael Kamp Asja Fischer and Martin Jaggi},
url = {https://openreview.net/pdf?id=LfmZh91tDI},
year = {2024},
date = {2024-05-07},
urldate = {2024-05-07},
booktitle = {International Conference on Learning Representations (ICLR)},
publisher = {Curran Associates, Inc},
abstract = {Averaging neural network parameters is an intuitive method for fusing the knowledge of two independent models. It is most prominently used in federated learning. If models are averaged at the end of training, this can only lead to a good performing model if the loss surface of interest is very particular, i.e., the loss in the exact middle between the two models needs to be sufficiently low. This is impossible to guarantee for the non-convex losses of state-of-the-art networks. For averaging models trained on vastly different datasets, it was proposed to average only the parameters of particular layers or combinations of layers, resulting in better performing models. To get a better understanding of the effect of layer-wise averaging, we analyse the performance of the models that result from averaging single layers, or groups of layers. Based on our empirical and theoretical investigation, we introduce a novel notion of the layer-wise linear connectivity, and show that deep networks do not have layer-wise barriers between them. We analyze additionally the layer-wise personalization averaging and conjecture that in particular problem setup all the partial aggregations result in the approximately same performance.},
keywords = {deep learning, layer-wise, linear mode connectivity},
pubstate = {published},
tppubtype = {inproceedings}
}
Yang, Fan; Bodic, Pierre Le; Kamp, Michael; Boley, Mario
Orthogonal Gradient Boosting for Interpretable Additive Rule Ensembles Proceedings Article
In: Proceedings of the 26th International Conference on Artificial Intelligence and Statistics (AISTATS), 2024.
Abstract | BibTeX | Tags: complexity, explainability, interpretability, interpretable, machine learning, rule ensemble, rule mining, XAI
@inproceedings{yang2024orthogonal,
title = {Orthogonal Gradient Boosting for Interpretable Additive Rule Ensembles},
author = {Fan Yang and Pierre Le Bodic and Michael Kamp and Mario Boley},
year = {2024},
date = {2024-05-02},
urldate = {2024-05-02},
booktitle = {Proceedings of the 26th International Conference on Artificial Intelligence and Statistics (AISTATS)},
abstract = {Gradient boosting of prediction rules is an efficient approach to learn potentially interpretable yet accurate probabilistic models. However, actual interpretability requires to limit the number and size of the generated rules, and existing boosting variants are not designed for this purpose. Though corrective boosting refits all rule weights in each iteration to minimise prediction risk, the included rule conditions tend to be sub-optimal, because commonly used objective functions fail to anticipate this refitting. Here, we address this issue by a new objective function that measures the angle between the risk gradient vector and the projection of the condition output vector onto the orthogonal complement of the already selected conditions. This approach correctly approximates the ideal update of adding the risk gradient itself to the model and favours the inclusion of more general and thus shorter rules. As we demonstrate using a wide range of prediction tasks, this significantly improves the comprehensibility/accuracy trade-off of the fitted ensemble. Additionally, we show how objective values for related rule conditions can be computed incrementally to avoid any substantial computational overhead of the new method.},
keywords = {complexity, explainability, interpretability, interpretable, machine learning, rule ensemble, rule mining, XAI},
pubstate = {published},
tppubtype = {inproceedings}
}
2023
Adilova, Linara; Abourayya, Amr; Li, Jianning; Dada, Amin; Petzka, Henning; Egger, Jan; Kleesiek, Jens; Kamp, Michael
FAM: Relative Flatness Aware Minimization Proceedings Article
In: Proceedings of the ICML Workshop on Topology, Algebra, and Geometry in Machine Learning (TAG-ML), 2023.
Links | BibTeX | Tags: deep learning, flatness, generalization, machine learning, relative flatness, theory of deep learning
@inproceedings{adilova2023fam,
title = {FAM: Relative Flatness Aware Minimization},
author = {Linara Adilova and Amr Abourayya and Jianning Li and Amin Dada and Henning Petzka and Jan Egger and Jens Kleesiek and Michael Kamp},
url = {https://michaelkamp.org/wp-content/uploads/2023/06/fam_regularization.pdf},
year = {2023},
date = {2023-07-22},
urldate = {2023-07-22},
booktitle = {Proceedings of the ICML Workshop on Topology, Algebra, and Geometry in Machine Learning (TAG-ML)},
keywords = {deep learning, flatness, generalization, machine learning, relative flatness, theory of deep learning},
pubstate = {published},
tppubtype = {inproceedings}
}
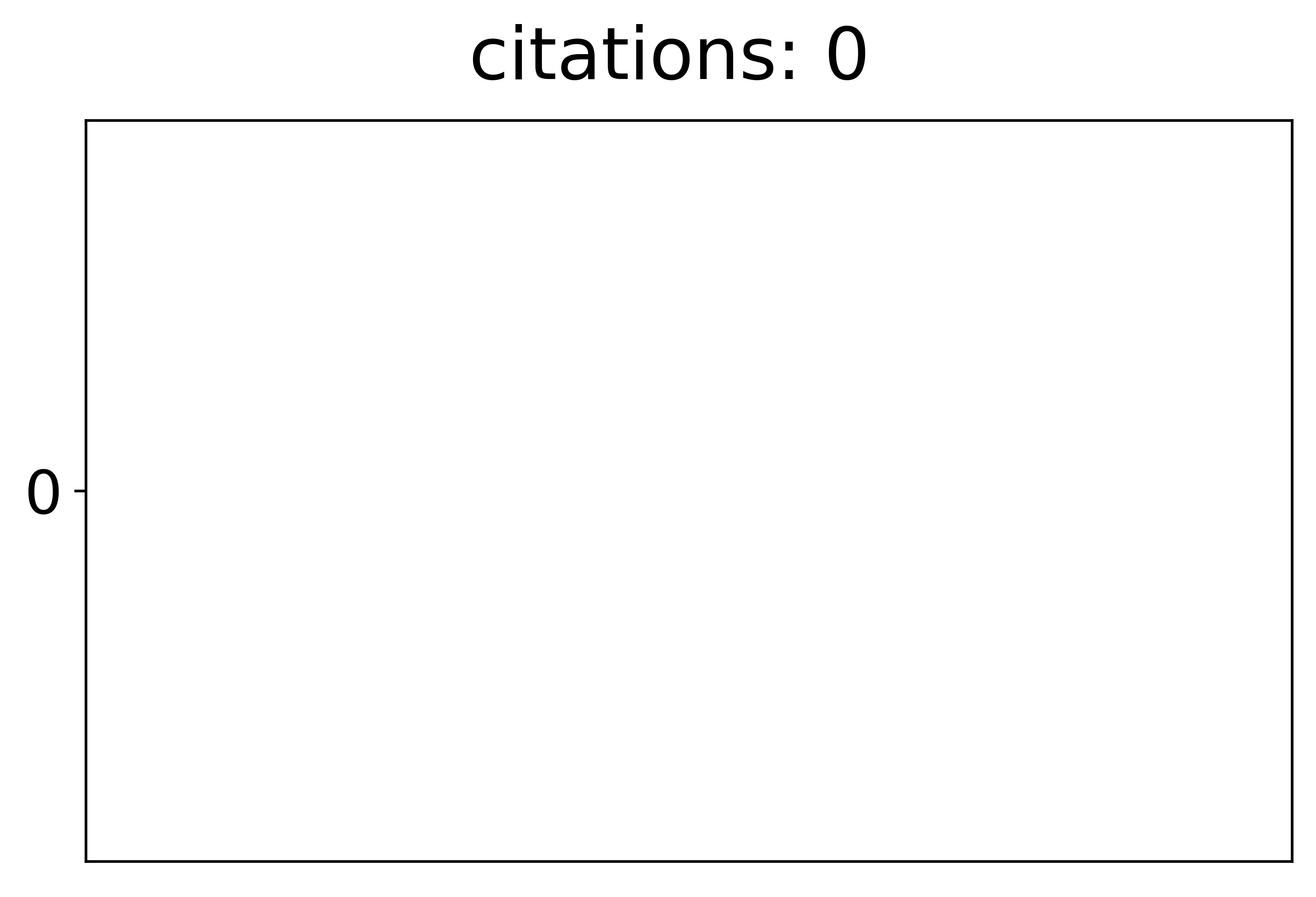
Michael Kamp Linara Adilova, Gennady Andrienko
Re-interpreting Rules Interpretability Journal Article
In: International Journal of Data Science and Analytics, 2023.
BibTeX | Tags: interpretable, machine learning, rule learning, XAI
@article{adilova2023reinterpreting,
title = {Re-interpreting Rules Interpretability},
author = {Linara Adilova, Michael Kamp, Gennady Andrienko, Natalia Andrienko},
year = {2023},
date = {2023-06-30},
urldate = {2023-06-30},
journal = {International Journal of Data Science and Analytics},
keywords = {interpretable, machine learning, rule learning, XAI},
pubstate = {published},
tppubtype = {article}
}
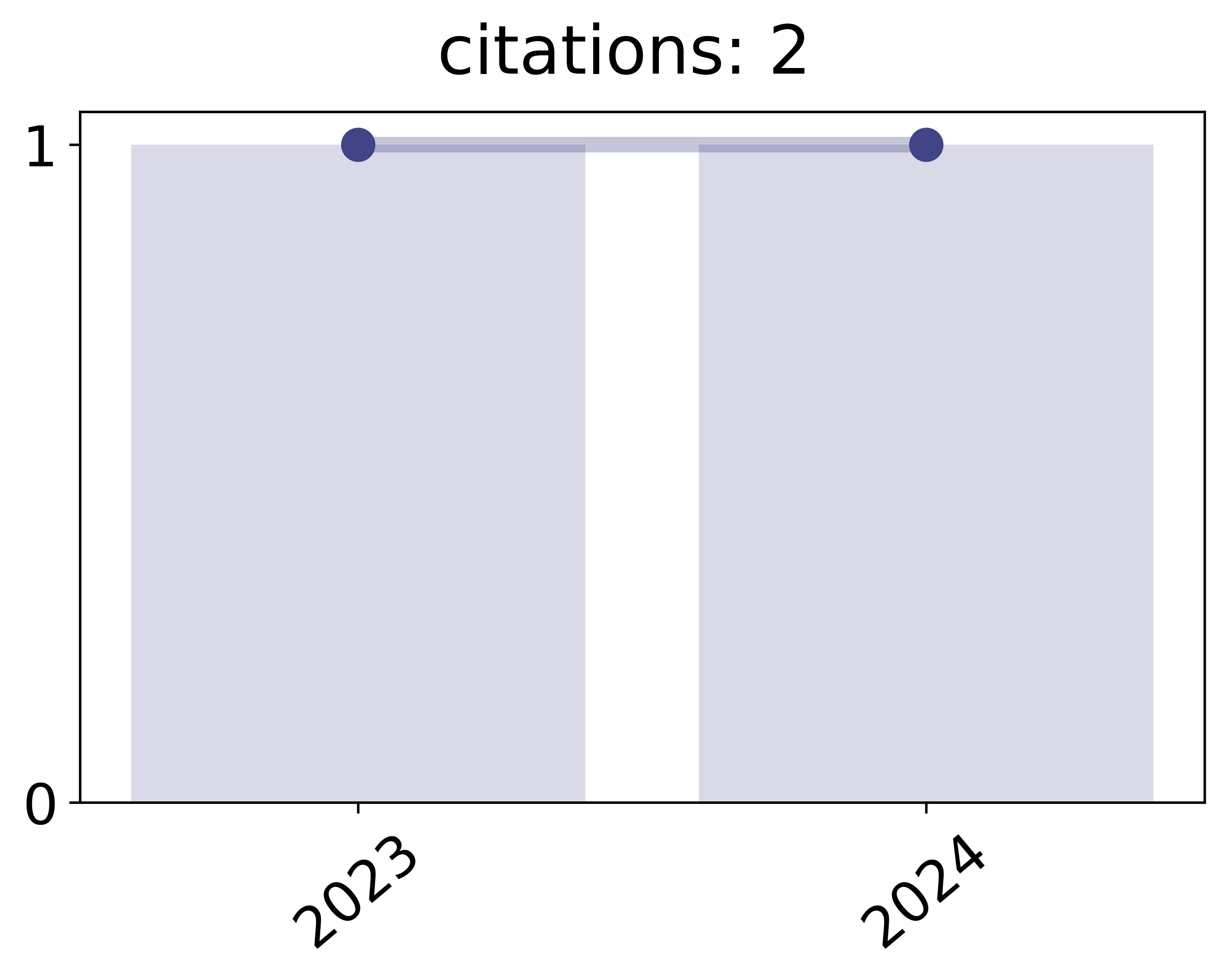
Kamp, Michael; Fischer, Jonas; Vreeken, Jilles
Federated Learning from Small Datasets Proceedings Article
In: International Conference on Learning Representations (ICLR), 2023.
Links | BibTeX | Tags: black-box, black-box parallelization, daisy, daisy-chaining, FedDC, federated learning, small, small datasets
@inproceedings{kamp2023federated,
title = {Federated Learning from Small Datasets},
author = {Michael Kamp and Jonas Fischer and Jilles Vreeken},
url = {https://michaelkamp.org/wp-content/uploads/2022/08/FederatedLearingSmallDatasets.pdf},
year = {2023},
date = {2023-05-01},
urldate = {2023-05-01},
booktitle = {International Conference on Learning Representations (ICLR)},
journal = {arXiv preprint arXiv:2110.03469},
keywords = {black-box, black-box parallelization, daisy, daisy-chaining, FedDC, federated learning, small, small datasets},
pubstate = {published},
tppubtype = {inproceedings}
}
David Kaltenpoth Osman Mian, Michael Kamp
Nothing but Regrets - Privacy-Preserving Federated Causal Discovery Proceedings Article
In: International Conference on Artificial Intelligence and Statistics (AISTATS), 2023.
BibTeX | Tags: causal discovery, causality, explainable, federated, federated causal discovery, federated learning, interpretable
@inproceedings{mian2022nothing,
title = {Nothing but Regrets - Privacy-Preserving Federated Causal Discovery},
author = {Osman Mian, David Kaltenpoth, Michael Kamp, Jilles Vreeken},
year = {2023},
date = {2023-04-25},
urldate = {2023-04-25},
booktitle = {International Conference on Artificial Intelligence and Statistics (AISTATS)},
keywords = {causal discovery, causality, explainable, federated, federated causal discovery, federated learning, interpretable},
pubstate = {published},
tppubtype = {inproceedings}
}
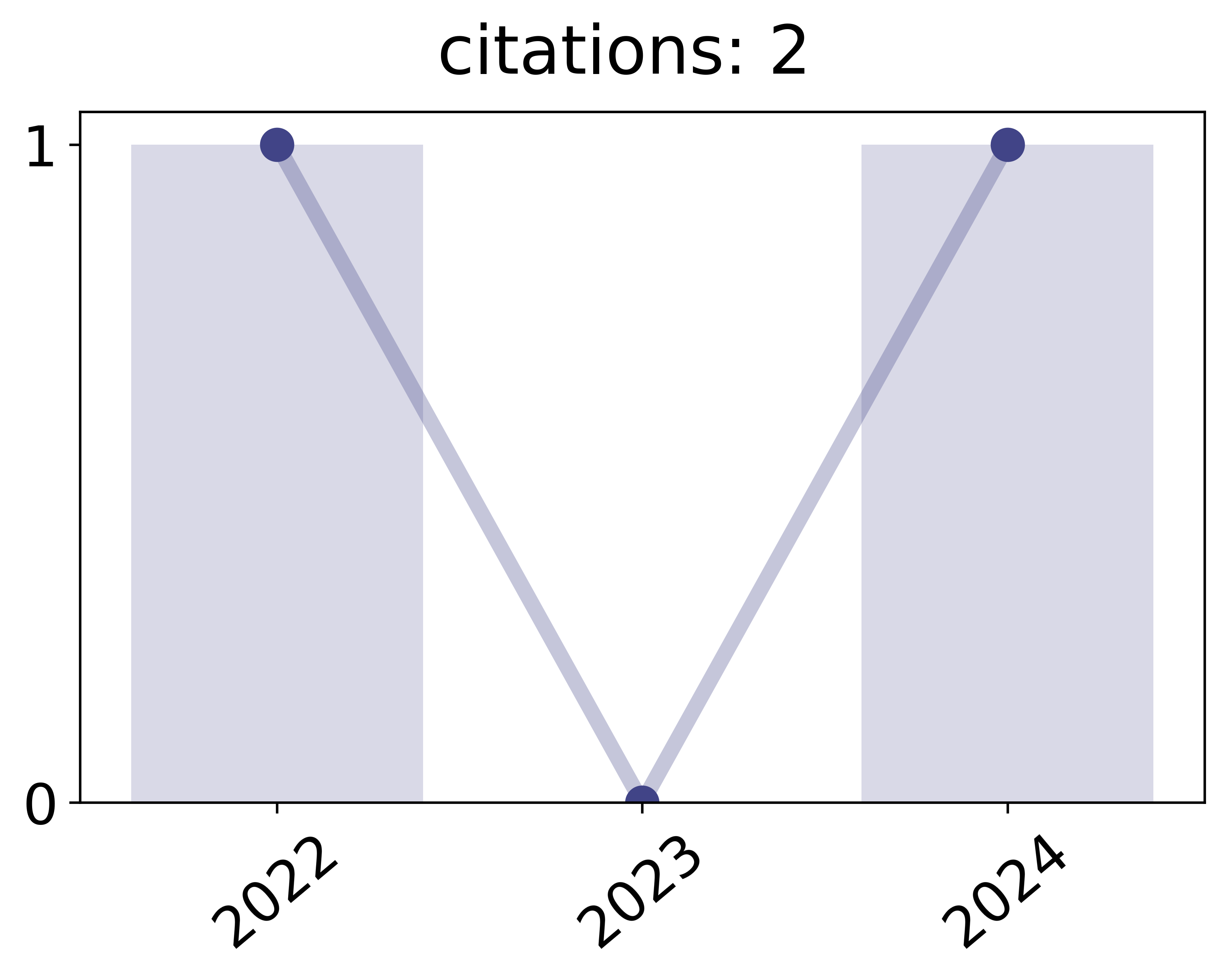
Mian, Osman; Kamp, Michael; Vreeken, Jilles
Information-Theoretic Causal Discovery and Intervention Detection over Multiple Environments Proceedings Article
In: Proceedings of the AAAI Conference on Artificial Intelligence (AAAI), 2023.
BibTeX | Tags: causal discovery, causality, federated, federated causal discovery, federated learning, intervention
@inproceedings{mian2023informationb,
title = {Information-Theoretic Causal Discovery and Intervention Detection over Multiple Environments},
author = {Osman Mian and Michael Kamp and Jilles Vreeken},
year = {2023},
date = {2023-02-07},
urldate = {2023-02-07},
booktitle = {Proceedings of the AAAI Conference on Artificial Intelligence (AAAI)},
keywords = {causal discovery, causality, federated, federated causal discovery, federated learning, intervention},
pubstate = {published},
tppubtype = {inproceedings}
}
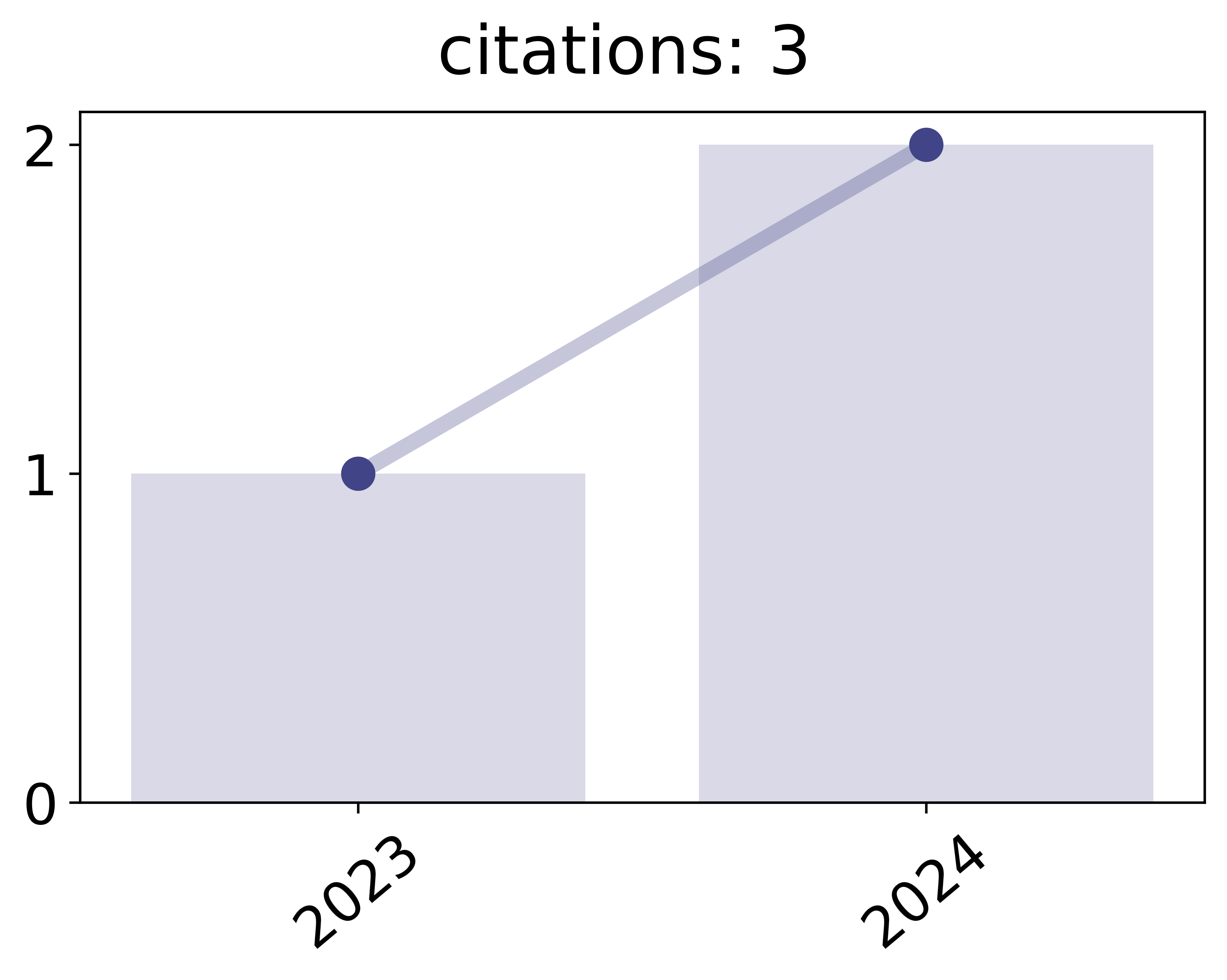
Li, Jianning; Ferreira, André; Puladi, Behrus; Alves, Victor; Kamp, Michael; Kim, Moon; Nensa, Felix; Kleesiek, Jens; Ahmadi, Seyed-Ahmad; Egger, Jan
Open-source skull reconstruction with MONAI Journal Article
In: SoftwareX, vol. 23, pp. 101432, 2023.
BibTeX | Tags:
@article{li2023open,
title = {Open-source skull reconstruction with MONAI},
author = {Jianning Li and André Ferreira and Behrus Puladi and Victor Alves and Michael Kamp and Moon Kim and Felix Nensa and Jens Kleesiek and Seyed-Ahmad Ahmadi and Jan Egger},
year = {2023},
date = {2023-01-01},
urldate = {2023-01-01},
journal = {SoftwareX},
volume = {23},
pages = {101432},
publisher = {Elsevier},
keywords = {},
pubstate = {published},
tppubtype = {article}
}
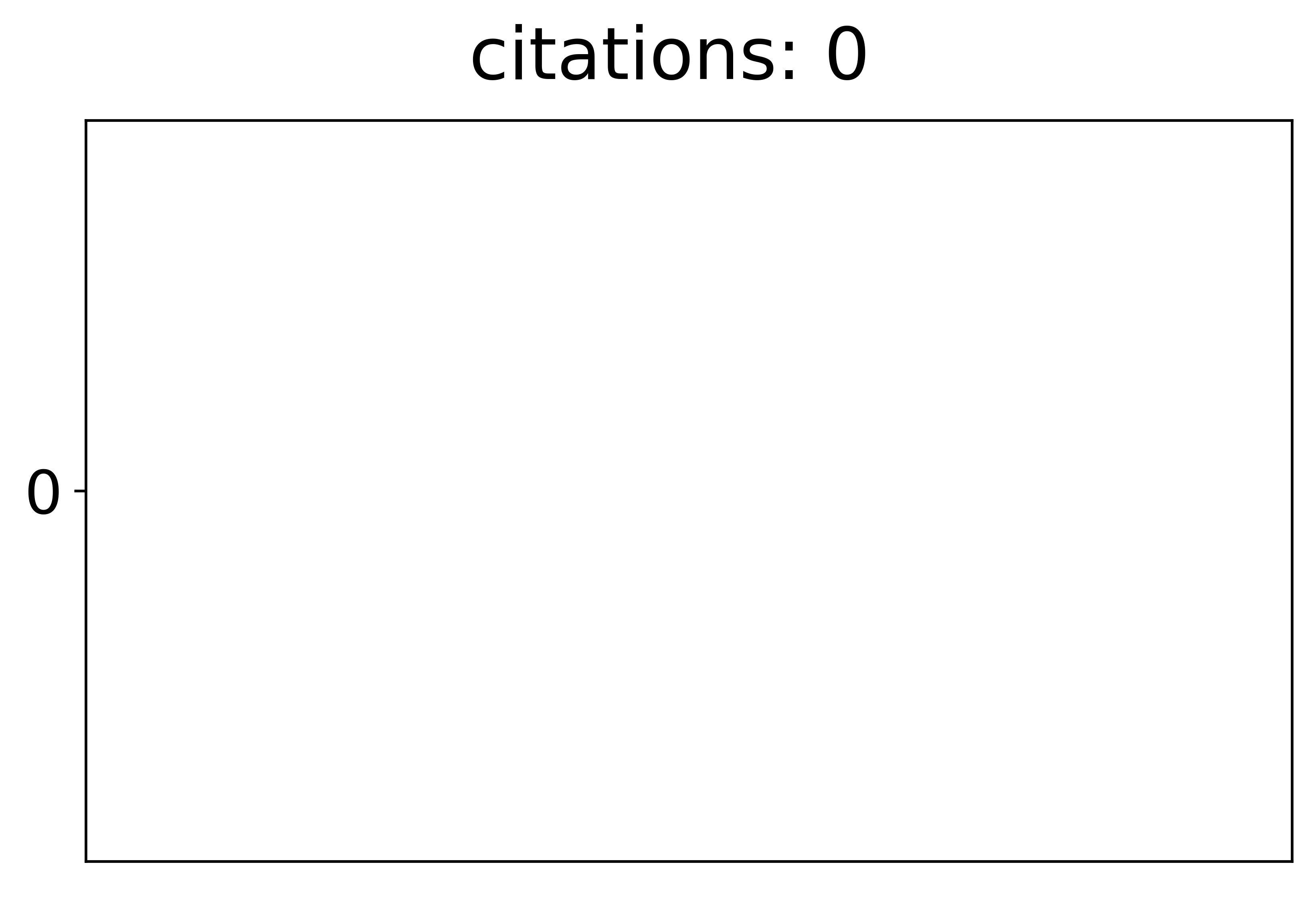
Adilova, Linara; Chen, Siming; Kamp, Michael
Informed Novelty Detection in Sequential Data by Per-Cluster Modeling Proceedings Article
In: ICML workshop on Artificial Intelligence & Human Computer Interaction, 2023.
@inproceedings{adilova2023informed,
title = {Informed Novelty Detection in Sequential Data by Per-Cluster Modeling},
author = {Linara Adilova and Siming Chen and Michael Kamp},
url = {https://michaelkamp.org/wp-content/uploads/2023/09/Informed_Novelty_Detection_in_Sequential_Data_by_Per_Cluster_Modeling.pdf},
year = {2023},
date = {2023-01-01},
urldate = {2023-01-01},
booktitle = {ICML workshop on Artificial Intelligence & Human Computer Interaction},
keywords = {},
pubstate = {published},
tppubtype = {inproceedings}
}
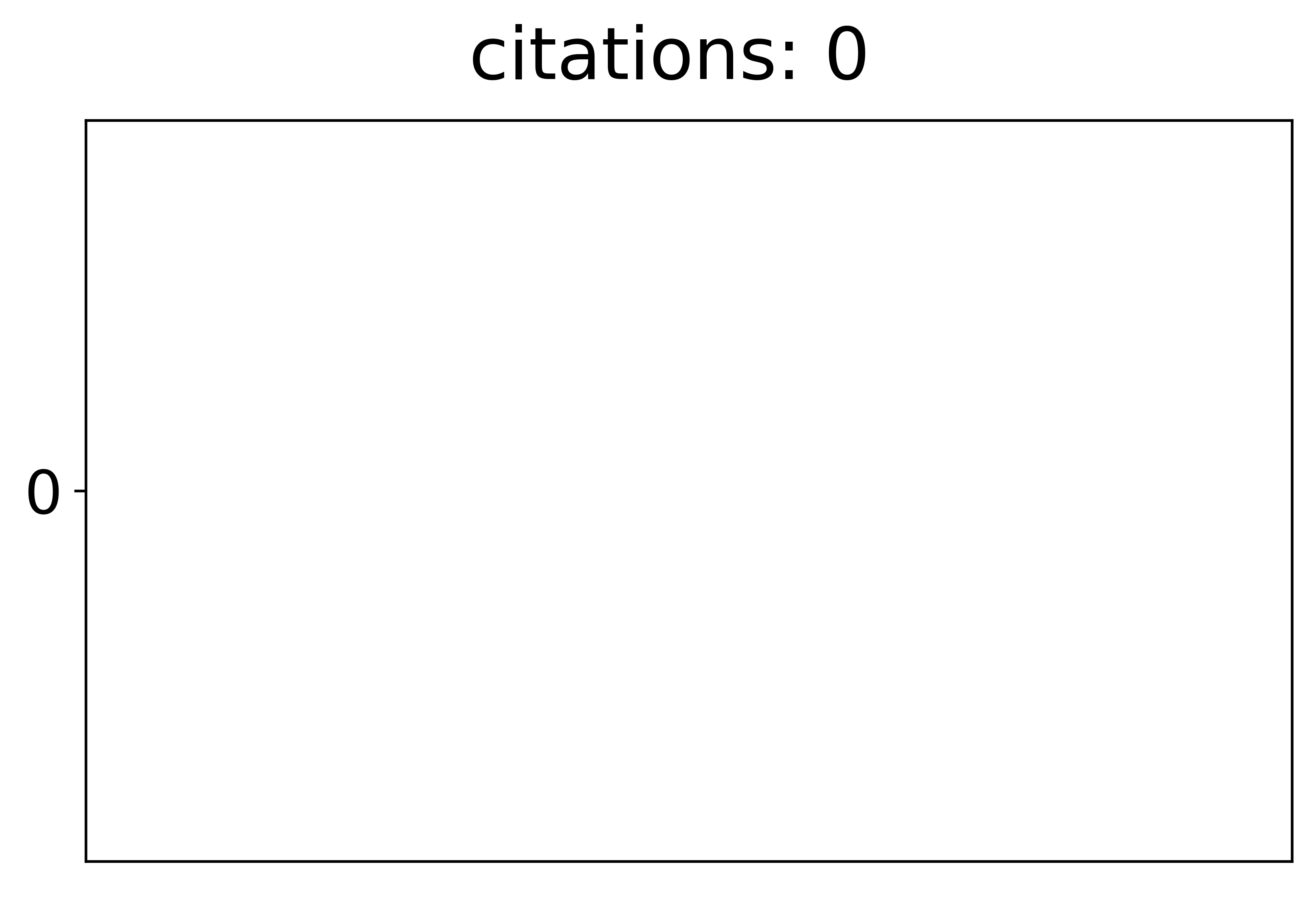
2022
Wang, Junhong; Li, Yun; Zhou, Zhaoyu; Wang, Chengshun; Hou, Yijie; Zhang, Li; Xue, Xiangyang; Kamp, Michael; Zhang, Xiaolong; Chen, Siming
When, Where and How does it fail? A Spatial-temporal Visual Analytics Approach for Interpretable Object Detection in Autonomous Driving Journal Article
In: IEEE Transactions on Visualization and Computer Graphics, 2022.
BibTeX | Tags:
@article{wang2022and,
title = {When, Where and How does it fail? A Spatial-temporal Visual Analytics Approach for Interpretable Object Detection in Autonomous Driving},
author = {Junhong Wang and Yun Li and Zhaoyu Zhou and Chengshun Wang and Yijie Hou and Li Zhang and Xiangyang Xue and Michael Kamp and Xiaolong Zhang and Siming Chen},
year = {2022},
date = {2022-01-01},
urldate = {2022-01-01},
journal = {IEEE Transactions on Visualization and Computer Graphics},
publisher = {IEEE},
keywords = {},
pubstate = {published},
tppubtype = {article}
}
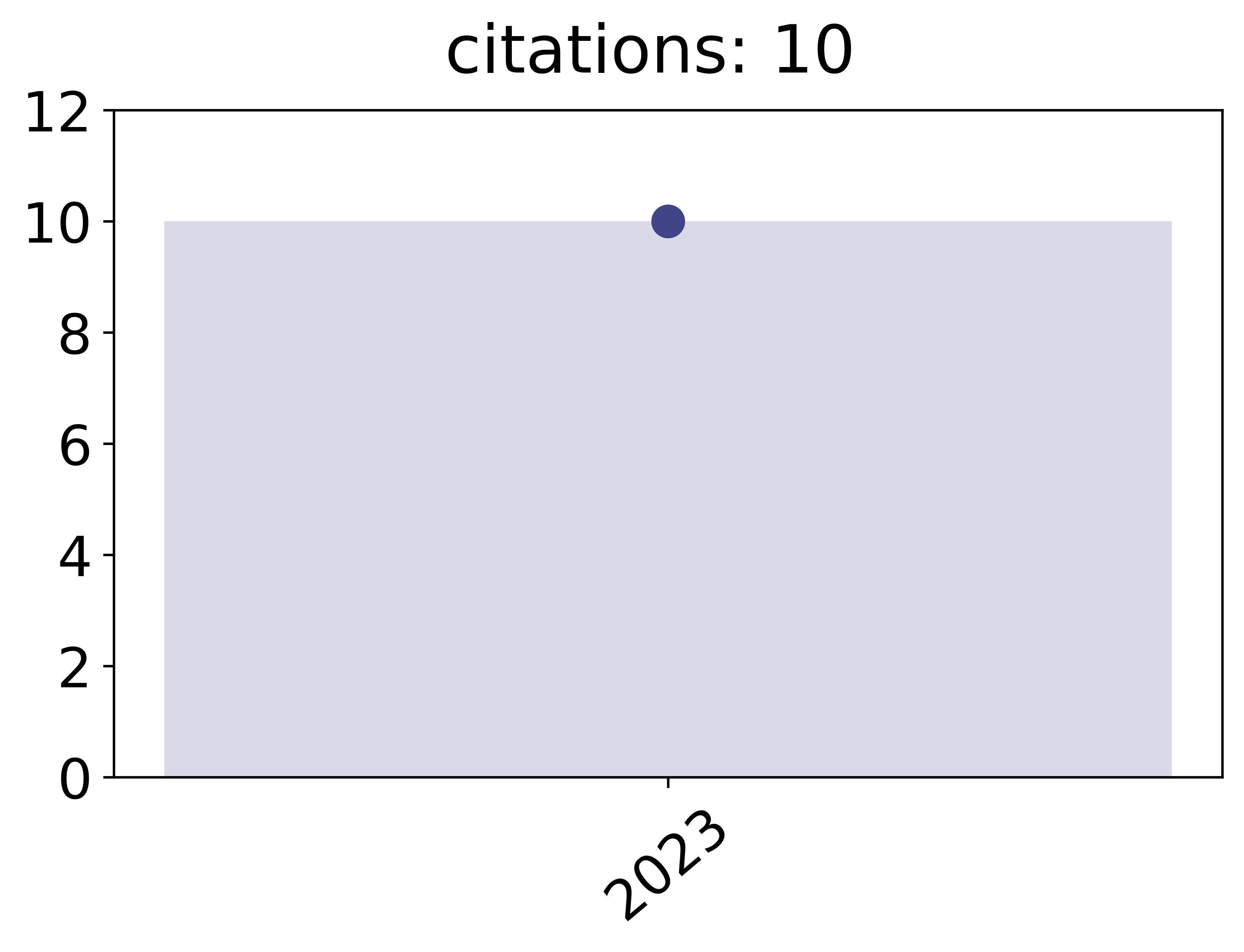
Mian, Osman; Kaltenpoth, David; Kamp, Michael
Regret-based Federated Causal Discovery Proceedings Article
In: The KDD'22 Workshop on Causal Discovery, pp. 61â69, PMLR 2022.
BibTeX | Tags:
@inproceedings{mian2022regret,
title = {Regret-based Federated Causal Discovery},
author = {Osman Mian and David Kaltenpoth and Michael Kamp},
year = {2022},
date = {2022-01-01},
urldate = {2022-01-01},
booktitle = {The KDD'22 Workshop on Causal Discovery},
pages = {61--69},
organization = {PMLR},
keywords = {},
pubstate = {published},
tppubtype = {inproceedings}
}
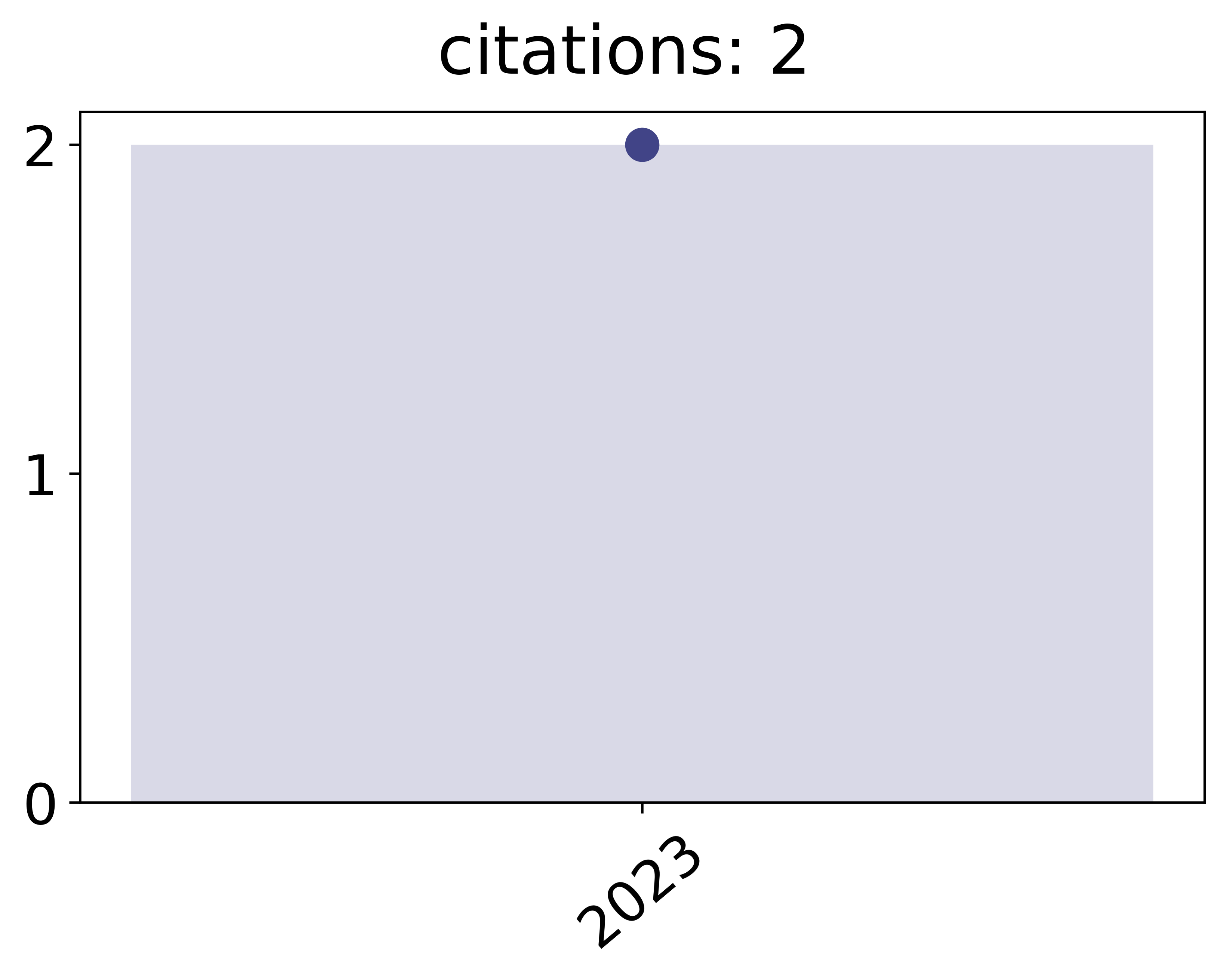
2021
Petzka, Henning; Kamp, Michael; Adilova, Linara; Sminchisescu, Cristian; Boley, Mario
Relative Flatness and Generalization Proceedings Article
In: Advances in Neural Information Processing Systems, Curran Associates, Inc., 2021.
Abstract | BibTeX | Tags: deep learning, flatness, generalization, Hessian, learning theory, relative flatness, theory of deep learning
@inproceedings{petzka2021relative,
title = {Relative Flatness and Generalization},
author = {Henning Petzka and Michael Kamp and Linara Adilova and Cristian Sminchisescu and Mario Boley},
year = {2021},
date = {2021-12-07},
urldate = {2021-12-07},
booktitle = {Advances in Neural Information Processing Systems},
publisher = {Curran Associates, Inc.},
abstract = {Flatness of the loss curve is conjectured to be connected to the generalization ability of machine learning models, in particular neural networks. While it has been empirically observed that flatness measures consistently correlate strongly with generalization, it is still an open theoretical problem why and under which circumstances flatness is connected to generalization, in particular in light of reparameterizations that change certain flatness measures but leave generalization unchanged. We investigate the connection between flatness and generalization by relating it to the interpolation from representative data, deriving notions of representativeness, and feature robustness. The notions allow us to rigorously connect flatness and generalization and to identify conditions under which the connection holds. Moreover, they give rise to a novel, but natural relative flatness measure that correlates strongly with generalization, simplifies to ridge regression for ordinary least squares, and solves the reparameterization issue.},
keywords = {deep learning, flatness, generalization, Hessian, learning theory, relative flatness, theory of deep learning},
pubstate = {published},
tppubtype = {inproceedings}
}
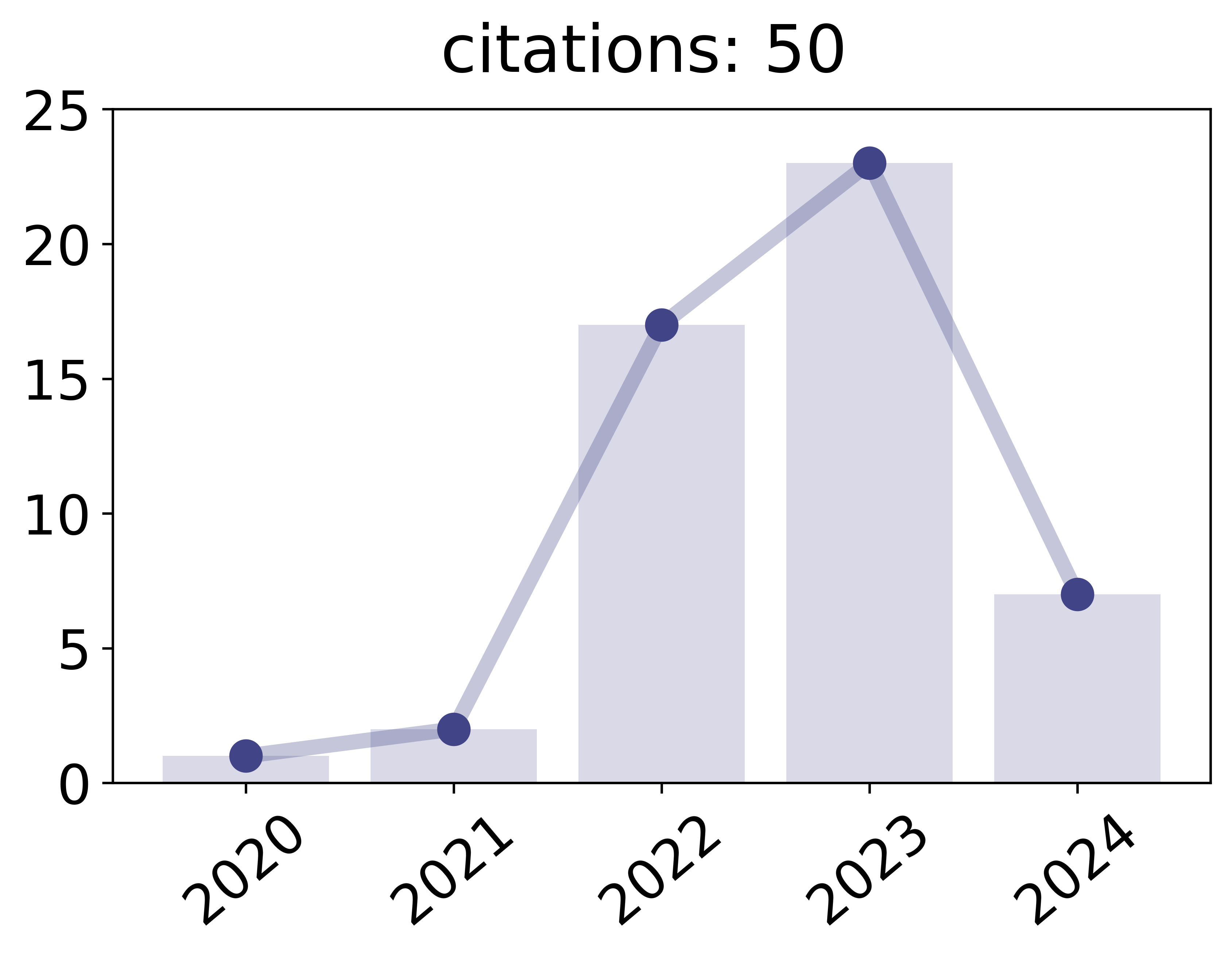
Linsner, Florian; Adilova, Linara; DĂ€ubener, Sina; Kamp, Michael; Fischer, Asja
Approaches to Uncertainty Quantification in Federated Deep Learning Workshop
Machine Learning and Principles and Practice of Knowledge Discovery in Databases: International Workshops of ECML PKDD 2021, vol. 2, Springer, 2021.
Links | BibTeX | Tags: federated learning, uncertainty
@workshop{linsner2021uncertainty,
title = {Approaches to Uncertainty Quantification in Federated Deep Learning},
author = {Florian Linsner and Linara Adilova and Sina DĂ€ubener and Michael Kamp and Asja Fischer},
url = {https://michaelkamp.org/wp-content/uploads/2022/04/federatedUncertainty.pdf},
year = {2021},
date = {2021-09-17},
urldate = {2021-09-17},
booktitle = {Machine Learning and Principles and Practice of Knowledge Discovery in Databases: International Workshops of ECML PKDD 2021},
issuetitle = {Workshop on Parallel, Distributed, and Federated Learning},
volume = {2},
pages = {128-145},
publisher = {Springer},
keywords = {federated learning, uncertainty},
pubstate = {published},
tppubtype = {workshop}
}
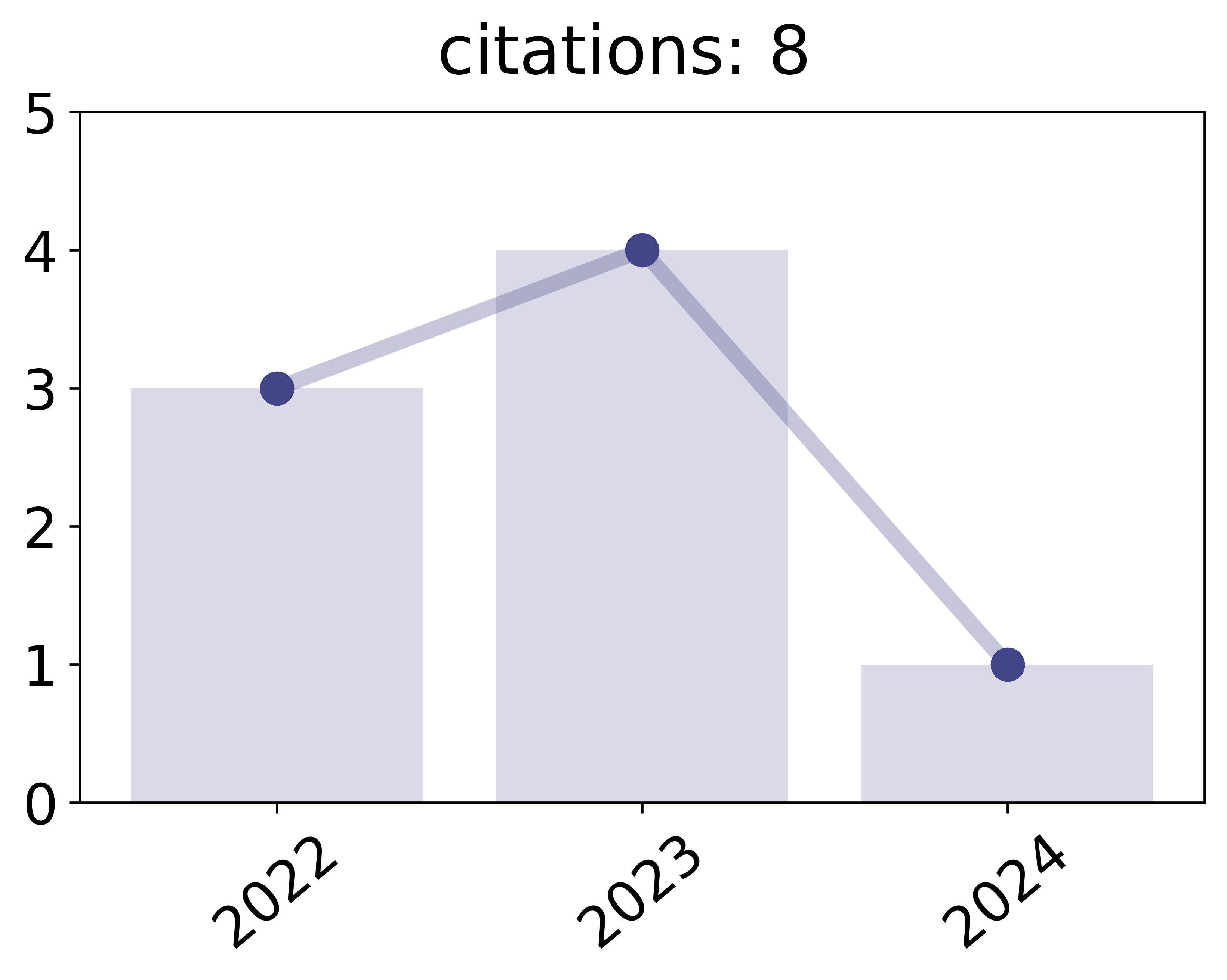
Li, Xiaoxiao; Jiang, Meirui; Zhang, Xiaofei; Kamp, Michael; Dou, Qi
FedBN: Federated Learning on Non-IID Features via Local Batch Normalization Proceedings Article
In: Proceedings of the 9th International Conference on Learning Representations (ICLR), 2021.
Abstract | Links | BibTeX | Tags: batch normalization, black-box parallelization, deep learning, federated learning
@inproceedings{li2021fedbn,
title = {FedBN: Federated Learning on Non-IID Features via Local Batch Normalization},
author = {Xiaoxiao Li and Meirui Jiang and Xiaofei Zhang and Michael Kamp and Qi Dou},
url = {https://michaelkamp.org/wp-content/uploads/2021/05/fedbn_federated_learning_on_non_iid_features_via_local_batch_normalization.pdf
https://michaelkamp.org/wp-content/uploads/2021/05/FedBN_appendix.pdf},
year = {2021},
date = {2021-05-03},
urldate = {2021-05-03},
booktitle = {Proceedings of the 9th International Conference on Learning Representations (ICLR)},
abstract = {The emerging paradigm of federated learning (FL) strives to enable collaborative training of deep models on the network edge without centrally aggregating raw data and hence improving data privacy. In most cases, the assumption of independent and identically distributed samples across local clients does not hold for federated learning setups. Under this setting, neural network training performance may vary significantly according to the data distribution and even hurt training convergence. Most of the previous work has focused on a difference in the distribution of labels or client shifts. Unlike those settings, we address an important problem of FL, e.g., different scanners/sensors in medical imaging, different scenery distribution in autonomous driving (highway vs. city), where local clients store examples with different distributions compared to other clients, which we denote as feature shift non-iid. In this work, we propose an effective method that uses local batch normalization to alleviate the feature shift before averaging models. The resulting scheme, called FedBN, outperforms both classical FedAvg, as well as the state-of-the-art for non-iid data (FedProx) on our extensive experiments. These empirical results are supported by a convergence analysis that shows in a simplified setting that FedBN has a faster convergence rate than FedAvg. Code is available at https://github.com/med-air/FedBN.},
keywords = {batch normalization, black-box parallelization, deep learning, federated learning},
pubstate = {published},
tppubtype = {inproceedings}
}
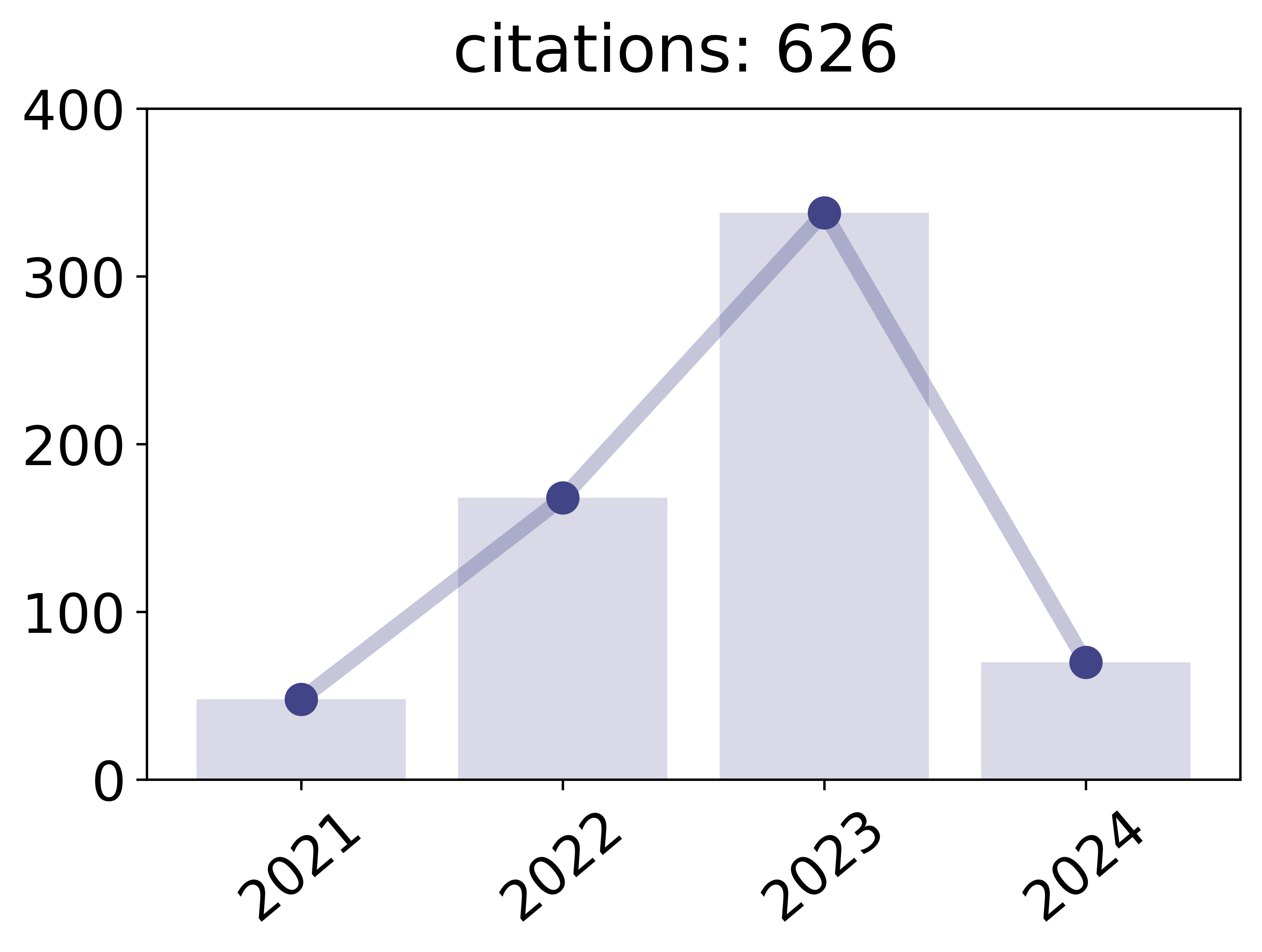
2020
Heppe, Lukas; Kamp, Michael; Adilova, Linara; Piatkowski, Nico; Heinrich, Danny; Morik, Katharina
Resource-Constrained On-Device Learning by Dynamic Averaging Workshop
Proceedings of the Workshop on Parallel, Distributed, and Federated Learning (PDFL) at ECMLPKDD, 2020.
Abstract | Links | BibTeX | Tags: black-box parallelization, distributed learning, edge computing, embedded, exponential family, FPGA, resource-efficient
@workshop{heppe2020resource,
title = {Resource-Constrained On-Device Learning by Dynamic Averaging},
author = {Lukas Heppe and Michael Kamp and Linara Adilova and Nico Piatkowski and Danny Heinrich and Katharina Morik},
url = {https://michaelkamp.org/wp-content/uploads/2020/10/Resource_Constrained_Federated_Learning-1.pdf},
year = {2020},
date = {2020-09-14},
urldate = {2020-09-14},
booktitle = {Proceedings of the Workshop on Parallel, Distributed, and Federated Learning (PDFL) at ECMLPKDD},
abstract = {The communication between data-generating devices is partially responsible for a growing portion of the worldâs power consumption. Thus reducing communication is vital, both, from an economical and an ecological perspective. For machine learning, on-device learning avoids sending raw data, which can reduce communication substantially. Furthermore, not centralizing the data protects privacy-sensitive data. However, most learning algorithms require hardware with high computation power and thus high energy consumption. In contrast, ultra-low-power processors, like FPGAs or micro-controllers, allow for energy-efficient learning of local models. Combined with communication-efficient distributed learning strategies, this reduces the overall energy consumption and enables applications that were yet impossible due to limited energy on local devices. The major challenge is then, that the low-power processors typically only have integer processing capabilities. This paper investigates an approach to communication-efficient on-device learning of integer exponential families that can be executed on low-power processors, is privacy-preserving, and effectively minimizes communication. The empirical evaluation shows that the approach can reach a model quality comparable to a centrally learned regular model with an order of magnitude less communication. Comparing the overall energy consumption, this reduces the required energy for solving the machine learning task by a significant amount.},
keywords = {black-box parallelization, distributed learning, edge computing, embedded, exponential family, FPGA, resource-efficient},
pubstate = {published},
tppubtype = {workshop}
}
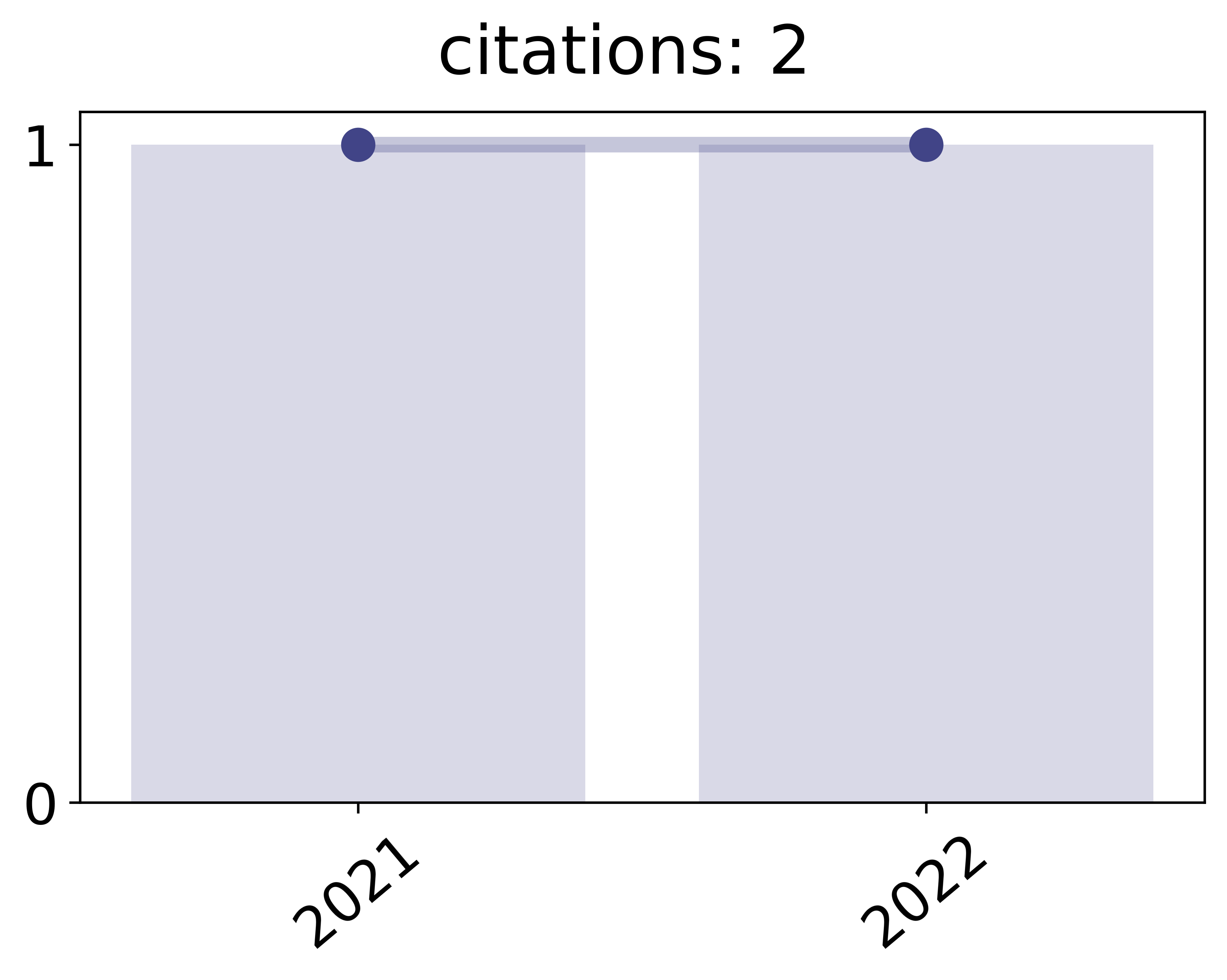
Petzka, Henning; Adilova, Linara; Kamp, Michael; Sminchisescu, Cristian
Feature-Robustness, Flatness and Generalization Error for Deep Neural Networks Workshop
2020.
Links | BibTeX | Tags: deep learning, flatness, generalization, learning theory, loss surface, neural networks, robustness
@workshop{petzka2020feature,
title = {Feature-Robustness, Flatness and Generalization Error for Deep Neural Networks},
author = {Henning Petzka and Linara Adilova and Michael Kamp and Cristian Sminchisescu},
url = {http://michaelkamp.org/wp-content/uploads/2020/01/flatnessFeatureRobustnessGeneralization.pdf},
year = {2020},
date = {2020-01-01},
urldate = {2020-01-01},
journal = {arXiv preprint arXiv:2001.00939},
keywords = {deep learning, flatness, generalization, learning theory, loss surface, neural networks, robustness},
pubstate = {published},
tppubtype = {workshop}
}
Welke, Pascal; Seiffarth, Florian; Kamp, Michael; Wrobel, Stefan
HOPS: Probabilistic Subtree Mining for Small and Large Graphs Proceedings Article
In: Proceedings of the 26th ACM SIGKDD International Conference on Knowledge Discovery & Data Mining, pp. 1275â1284, Association for Computing Machinery, Virtual Event, CA, USA, 2020, ISBN: 9781450379984.
Abstract | Links | BibTeX | Tags:
@inproceedings{10.1145/3394486.3403180,
title = {HOPS: Probabilistic Subtree Mining for Small and Large Graphs},
author = {Pascal Welke and Florian Seiffarth and Michael Kamp and Stefan Wrobel},
url = {https://doi.org/10.1145/3394486.3403180},
doi = {10.1145/3394486.3403180},
isbn = {9781450379984},
year = {2020},
date = {2020-01-01},
urldate = {2020-01-01},
booktitle = {Proceedings of the 26th ACM SIGKDD International Conference on Knowledge Discovery & Data Mining},
pages = {1275â1284},
publisher = {Association for Computing Machinery},
address = {Virtual Event, CA, USA},
series = {KDD '20},
abstract = {Frequent subgraph mining, i.e., the identification of relevant patterns in graph databases, is a well-known data mining problem with high practical relevance, since next to summarizing the data, the resulting patterns can also be used to define powerful domain-specific similarity functions for prediction. In recent years, significant progress has been made towards subgraph mining algorithms that scale to complex graphs by focusing on tree patterns and probabilistically allowing a small amount of incompleteness in the result. Nonetheless, the complexity of the pattern matching component used for deciding subtree isomorphism on arbitrary graphs has significantly limited the scalability of existing approaches. In this paper, we adapt sampling techniques from mathematical combinatorics to the problem of probabilistic subtree mining in arbitrary databases of many small to medium-size graphs or a single large graph. By restricting on tree patterns, we provide an algorithm that approximately counts or decides subtree isomorphism for arbitrary transaction graphs in sub-linear time with one-sided error. Our empirical evaluation on a range of benchmark graph datasets shows that the novel algorithm substantially outperforms state-of-the-art approaches both in the task of approximate counting of embeddings in single large graphs and in probabilistic frequent subtree mining in large databases of small to medium sized graphs.},
keywords = {},
pubstate = {published},
tppubtype = {inproceedings}
}
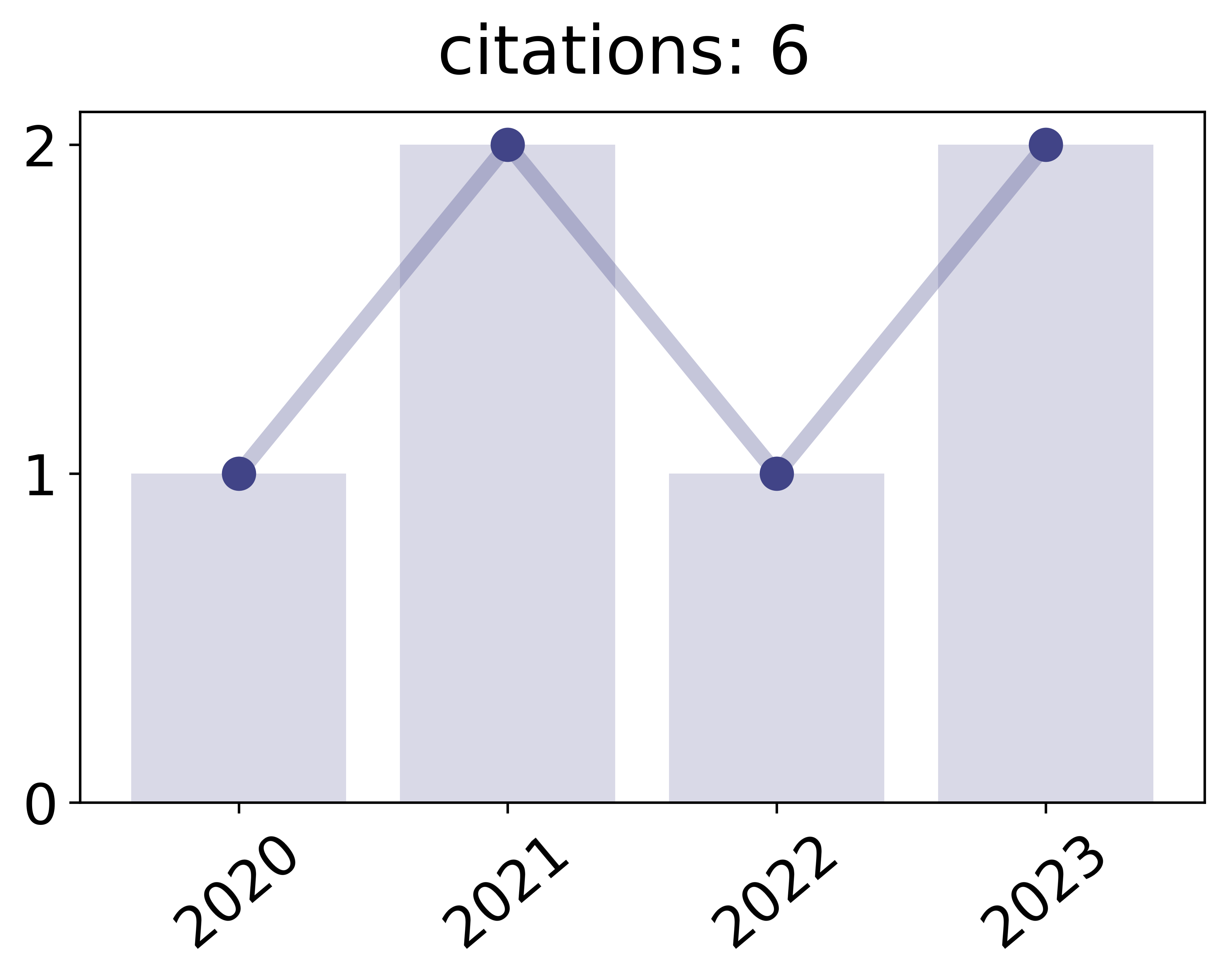
2019
Kamp, Michael
Black-Box Parallelization for Machine Learning PhD Thesis
UniversitÀts-und Landesbibliothek Bonn, 2019.
Abstract | Links | BibTeX | Tags: averaging, black-box, communication-efficient, convex optimization, deep learning, distributed, dynamic averaging, federated, learning theory, machine learning, parallelization, privacy, radon machine
@phdthesis{kamp2019black,
title = {Black-Box Parallelization for Machine Learning},
author = {Michael Kamp},
url = {https://d-nb.info/1200020057/34},
year = {2019},
date = {2019-01-01},
urldate = {2019-01-01},
school = {UniversitÀts-und Landesbibliothek Bonn},
abstract = {The landscape of machine learning applications is changing rapidly: large centralized datasets are replaced by high volume, high velocity data streams generated by a vast number of geographically distributed, loosely connected devices, such as mobile phones, smart sensors, autonomous vehicles or industrial machines. Current learning approaches centralize the data and process it in parallel in a cluster or computing center. This has three major disadvantages: (i) it does not scale well with the number of data-generating devices since their growth exceeds that of computing centers, (ii) the communication costs for centralizing the data are prohibitive in many applications, and (iii) it requires sharing potentially privacy-sensitive data. Pushing computation towards the data-generating devices alleviates these problems and allows to employ their otherwise unused computing power. However, current parallel learning approaches are designed for tightly integrated systems with low latency and high bandwidth, not for loosely connected distributed devices. Therefore, I propose a new paradigm for parallelization that treats the learning algorithm as a black box, training local models on distributed devices and aggregating them into a single strong one. Since this requires only exchanging models instead of actual data, the approach is highly scalable, communication-efficient, and privacy-preserving.
Following this paradigm, this thesis develops black-box parallelizations for two broad classes of learning algorithms. One approach can be applied to incremental learning algorithms, i.e., those that improve a model in iterations. Based on the utility of aggregations it schedules communication dynamically, adapting it to the hardness of the learning problem. In practice, this leads to a reduction in communication by orders of magnitude. It is analyzed for (i) online learning, in particular in the context of in-stream learning, which allows to guarantee optimal regret and for (ii) batch learning based on empirical risk minimization where optimal convergence can be guaranteed. The other approach is applicable to non-incremental algorithms as well. It uses a novel aggregation method based on the Radon point that allows to achieve provably high model quality with only a single aggregation. This is achieved in polylogarithmic runtime on quasi-polynomially many processors. This relates parallel machine learning to Nickâs class of parallel decision problems and is a step towards answering a fundamental open problem about the abilities and limitations of efficient parallel learning algorithms. An empirical study on real distributed systems confirms the potential of the approaches in realistic application scenarios.},
keywords = {averaging, black-box, communication-efficient, convex optimization, deep learning, distributed, dynamic averaging, federated, learning theory, machine learning, parallelization, privacy, radon machine},
pubstate = {published},
tppubtype = {phdthesis}
}
Following this paradigm, this thesis develops black-box parallelizations for two broad classes of learning algorithms. One approach can be applied to incremental learning algorithms, i.e., those that improve a model in iterations. Based on the utility of aggregations it schedules communication dynamically, adapting it to the hardness of the learning problem. In practice, this leads to a reduction in communication by orders of magnitude. It is analyzed for (i) online learning, in particular in the context of in-stream learning, which allows to guarantee optimal regret and for (ii) batch learning based on empirical risk minimization where optimal convergence can be guaranteed. The other approach is applicable to non-incremental algorithms as well. It uses a novel aggregation method based on the Radon point that allows to achieve provably high model quality with only a single aggregation. This is achieved in polylogarithmic runtime on quasi-polynomially many processors. This relates parallel machine learning to Nickâs class of parallel decision problems and is a step towards answering a fundamental open problem about the abilities and limitations of efficient parallel learning algorithms. An empirical study on real distributed systems confirms the potential of the approaches in realistic application scenarios.
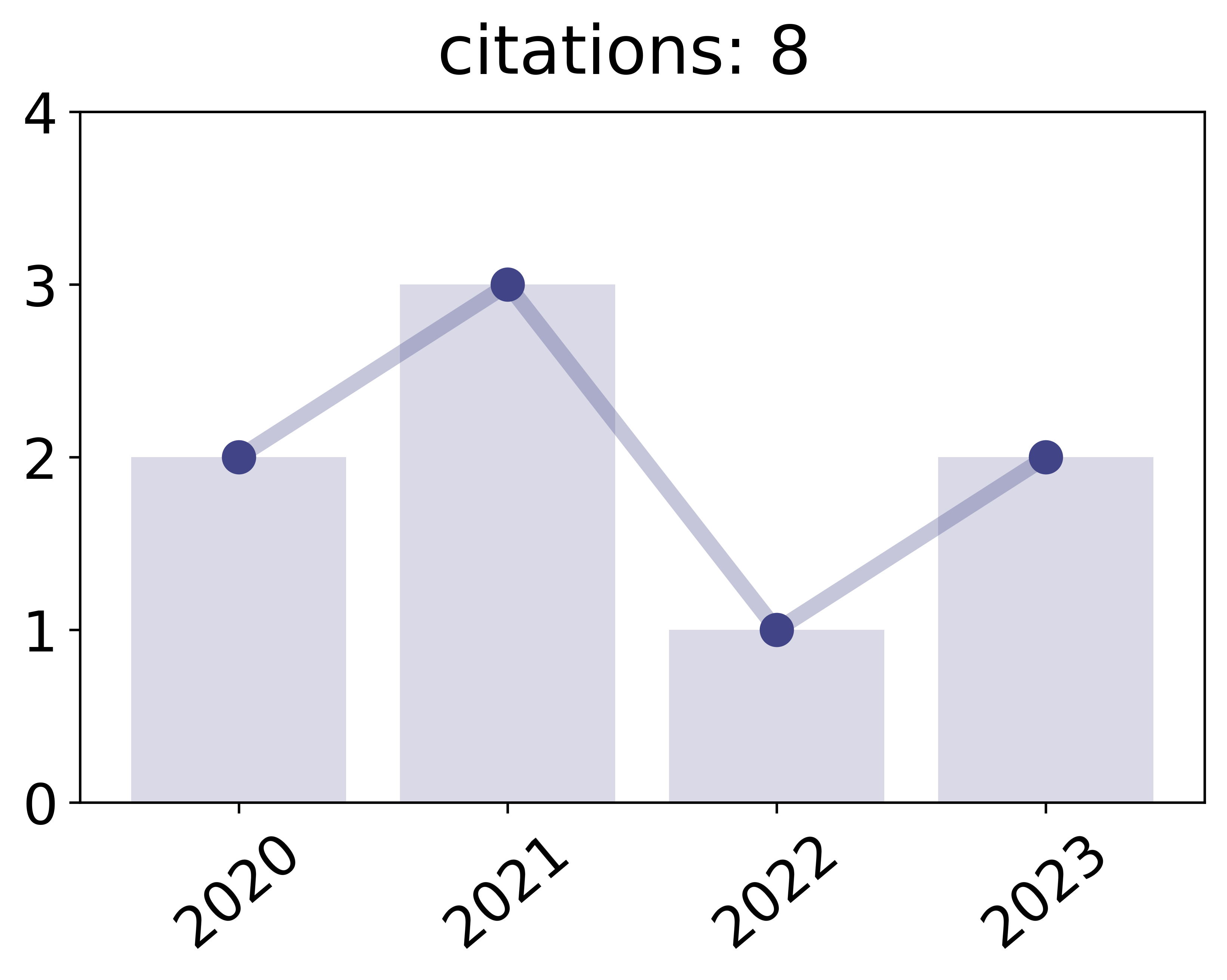
Adilova, Linara; Natious, Livin; Chen, Siming; Thonnard, Olivier; Kamp, Michael
System Misuse Detection via Informed Behavior Clustering and Modeling Workshop
2019 49th Annual IEEE/IFIP International Conference on Dependable Systems and Networks Workshops (DSN-W), IEEE 2019.
Links | BibTeX | Tags: anomaly detection, cybersecurity, DiSIEM, security, user behavior modelling, visualization
@workshop{adilova2019system,
title = {System Misuse Detection via Informed Behavior Clustering and Modeling},
author = {Linara Adilova and Livin Natious and Siming Chen and Olivier Thonnard and Michael Kamp},
url = {https://arxiv.org/pdf/1907.00874},
year = {2019},
date = {2019-01-01},
urldate = {2019-01-01},
booktitle = {2019 49th Annual IEEE/IFIP International Conference on Dependable Systems and Networks Workshops (DSN-W)},
pages = {15--23},
organization = {IEEE},
keywords = {anomaly detection, cybersecurity, DiSIEM, security, user behavior modelling, visualization},
pubstate = {published},
tppubtype = {workshop}
}
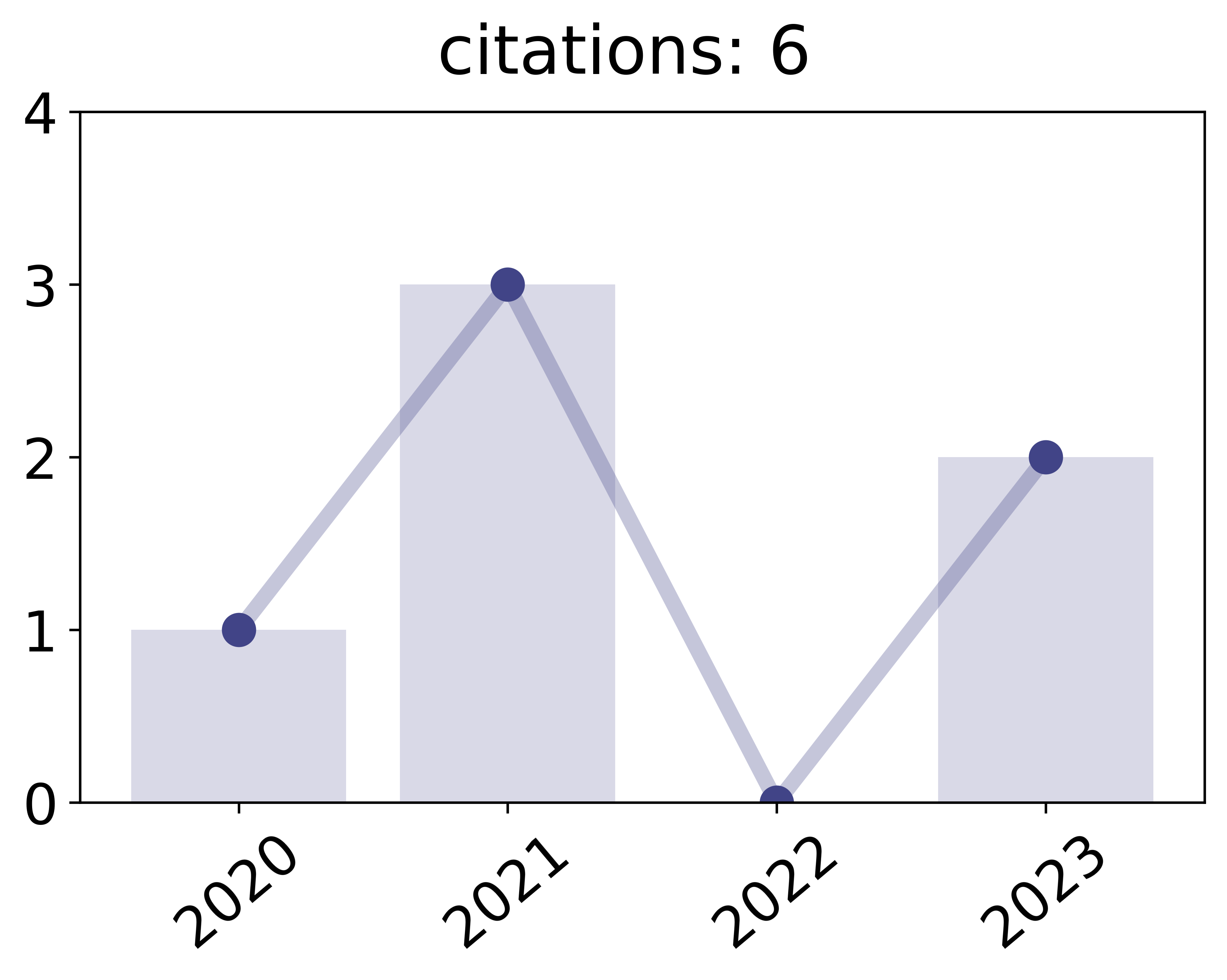
Petzka, Henning; Adilova, Linara; Kamp, Michael; Sminchisescu, Cristian
A Reparameterization-Invariant Flatness Measure for Deep Neural Networks Workshop
Science meets Engineering of Deep Learning workshop at NeurIPS, 2019.
Links | BibTeX | Tags: deep learning, flatness, generalization, learning theory, loss surface, neural networks, robustness
@workshop{petzka2019reparameterization,
title = {A Reparameterization-Invariant Flatness Measure for Deep Neural Networks},
author = {Henning Petzka and Linara Adilova and Michael Kamp and Cristian Sminchisescu},
url = {https://arxiv.org/pdf/1912.00058},
year = {2019},
date = {2019-01-01},
urldate = {2019-01-01},
booktitle = {Science meets Engineering of Deep Learning workshop at NeurIPS},
keywords = {deep learning, flatness, generalization, learning theory, loss surface, neural networks, robustness},
pubstate = {published},
tppubtype = {workshop}
}
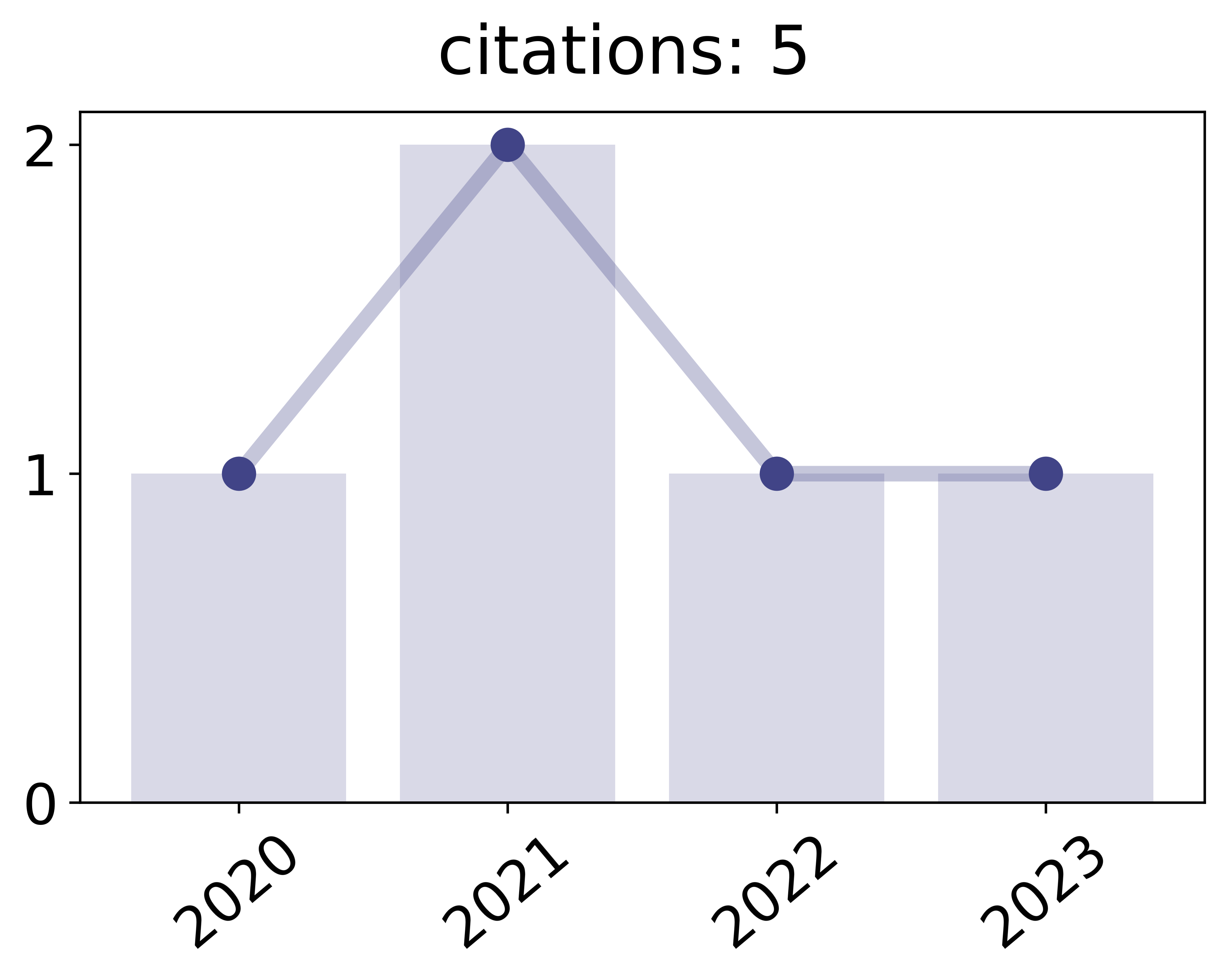
Adilova, Linara; Rosenzweig, Julia; Kamp, Michael
Information Theoretic Perspective of Federated Learning Workshop
NeurIPS Workshop on Information Theory and Machine Learning, 2019.
@workshop{adilova2019information,
title = {Information Theoretic Perspective of Federated Learning},
author = {Linara Adilova and Julia Rosenzweig and Michael Kamp},
url = {https://arxiv.org/pdf/1911.07652},
year = {2019},
date = {2019-01-01},
urldate = {2019-01-01},
booktitle = {NeurIPS Workshop on Information Theory and Machine Learning},
keywords = {},
pubstate = {published},
tppubtype = {workshop}
}
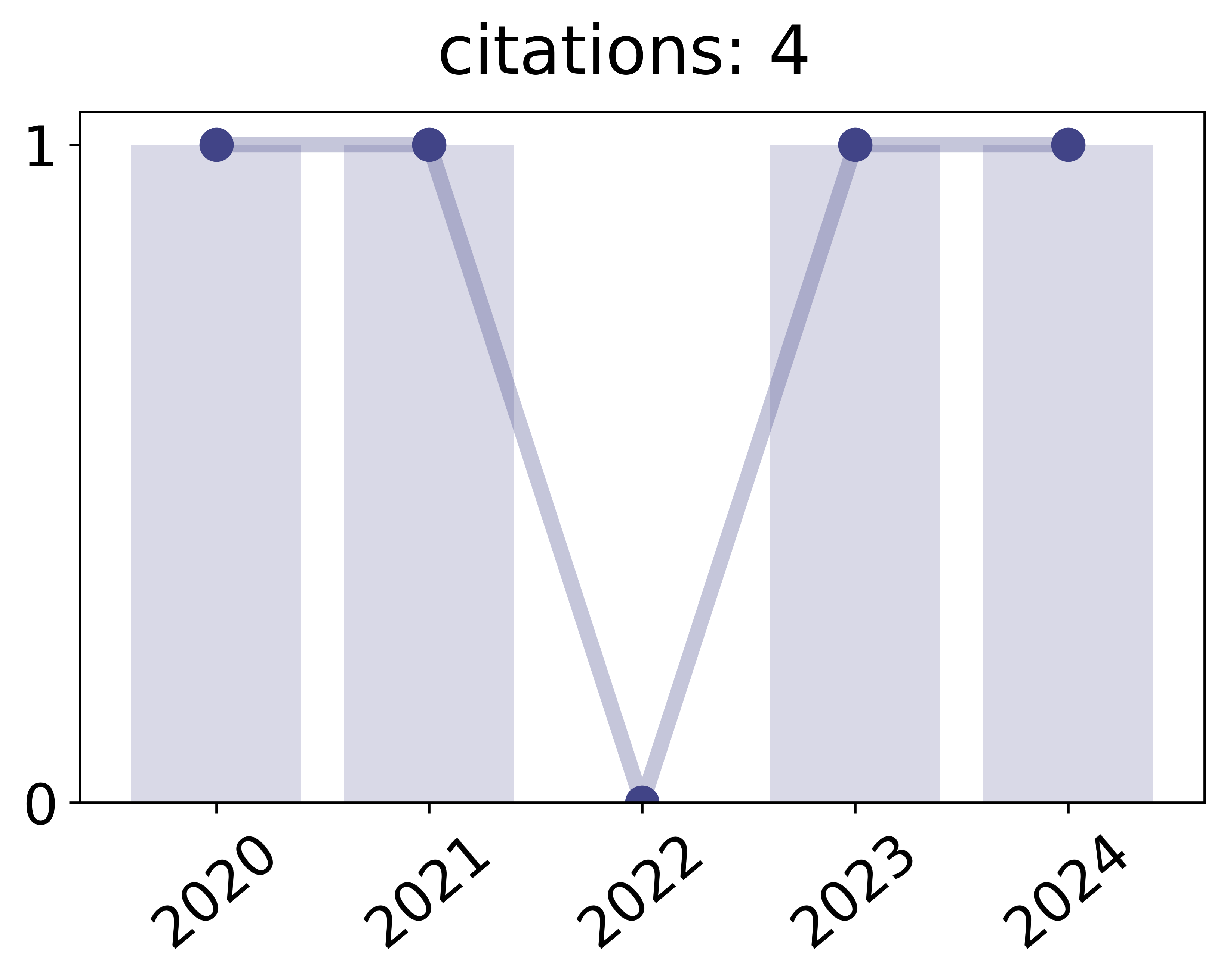
2018
Giesselbach, Sven; Ullrich, Katrin; Kamp, Michael; Paurat, Daniel; GĂ€rtner, Thomas
Corresponding Projections for Orphan Screening Workshop
Proceedings of the ML4H workshop at NeurIPS, 2018.
Links | BibTeX | Tags: corresponding projections, transfer learning, unsupervised
@workshop{giesselbach2018corresponding,
title = {Corresponding Projections for Orphan Screening},
author = {Sven Giesselbach and Katrin Ullrich and Michael Kamp and Daniel Paurat and Thomas GĂ€rtner},
url = {http://michaelkamp.org/wp-content/uploads/2018/12/cpNIPS.pdf},
year = {2018},
date = {2018-12-08},
urldate = {2018-12-08},
booktitle = {Proceedings of the ML4H workshop at NeurIPS},
keywords = {corresponding projections, transfer learning, unsupervised},
pubstate = {published},
tppubtype = {workshop}
}
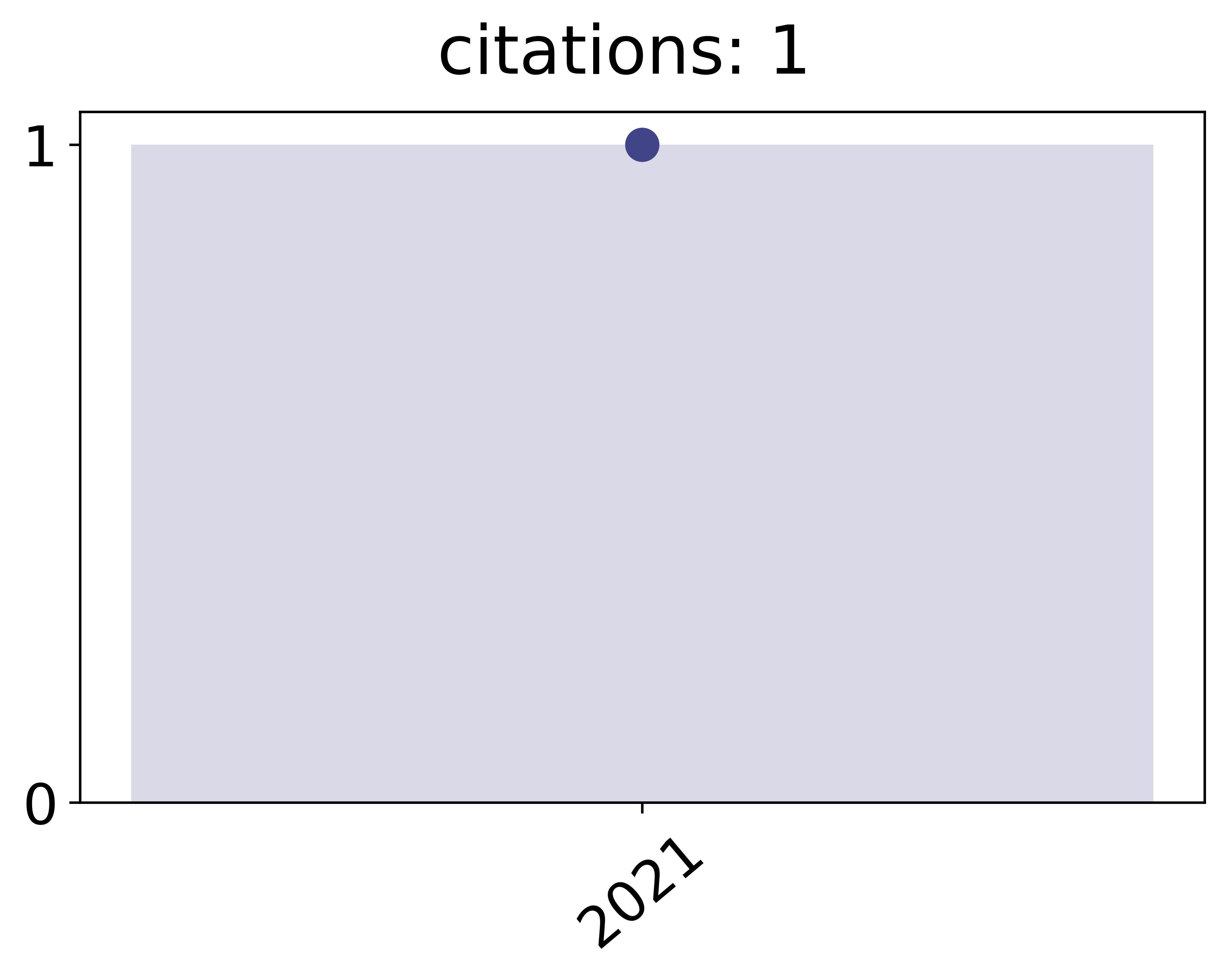
Nguyen, Phong H.; Chen, Siming; Andrienko, Natalia; Kamp, Michael; Adilova, Linara; Andrienko, Gennady; Thonnard, Olivier; Bessani, Alysson; Turkay, Cagatay
Designing Visualisation Enhancements for SIEM Systems Workshop
15th IEEE Symposium on Visualization for Cyber Security â VizSec, 2018.
Links | BibTeX | Tags: DiSIEM, SIEM, visual analytics, visualization
@workshop{phong2018designing,
title = {Designing Visualisation Enhancements for SIEM Systems},
author = {Phong H. Nguyen and Siming Chen and Natalia Andrienko and Michael Kamp and Linara Adilova and Gennady Andrienko and Olivier Thonnard and Alysson Bessani and Cagatay Turkay},
url = {http://michaelkamp.org/vizsec2018-poster-designing-visualisation-enhancements-for-siem-systems/},
year = {2018},
date = {2018-10-22},
urldate = {2018-10-22},
booktitle = {15th IEEE Symposium on Visualization for Cyber Security â VizSec},
keywords = {DiSIEM, SIEM, visual analytics, visualization},
pubstate = {published},
tppubtype = {workshop}
}
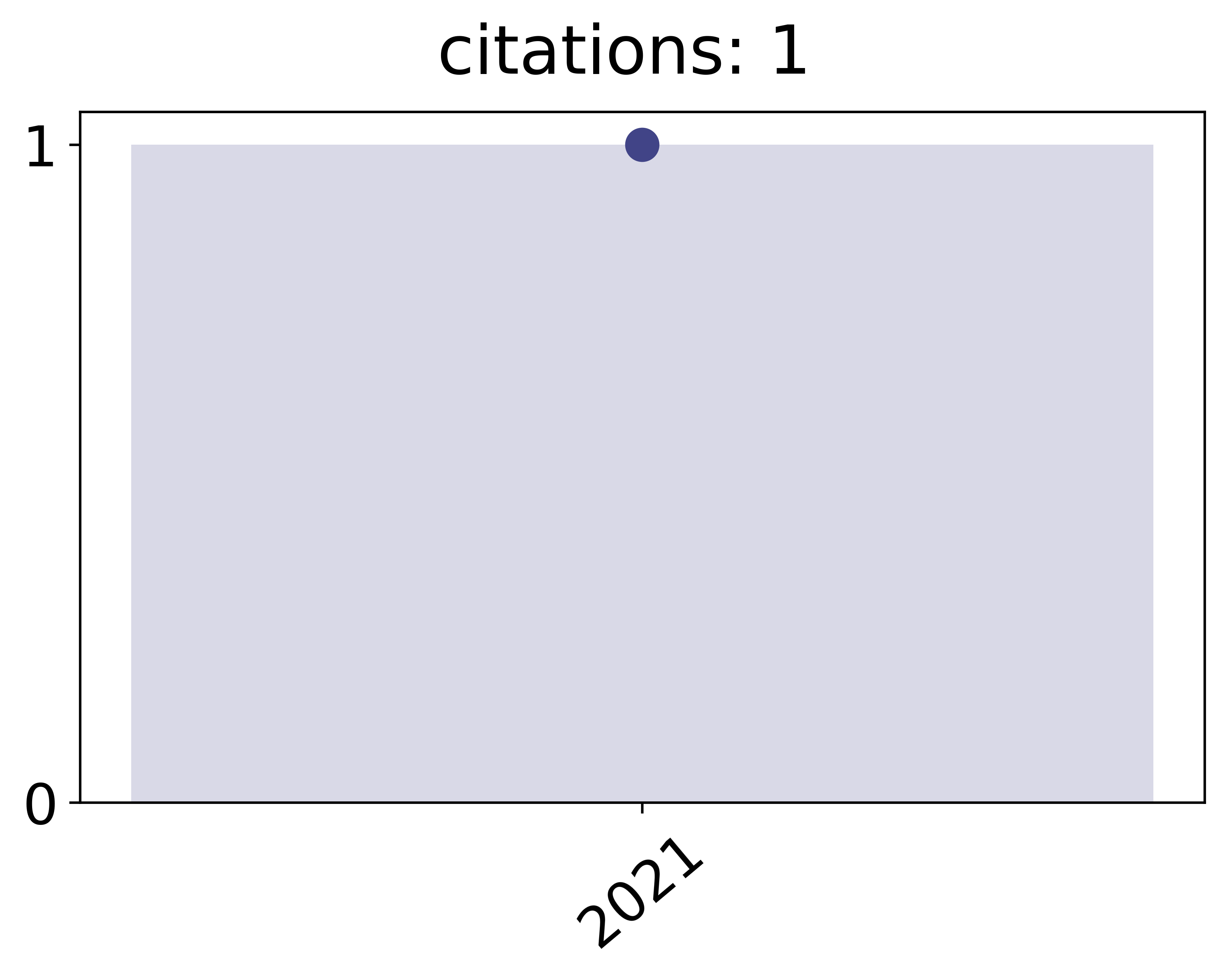
Kamp, Michael; Adilova, Linara; Sicking, Joachim; HĂŒger, Fabian; Schlicht, Peter; Wirtz, Tim; Wrobel, Stefan
Efficient Decentralized Deep Learning by Dynamic Model Averaging Proceedings Article
In: Machine Learning and Knowledge Discovery in Databases, Springer, 2018.
Abstract | Links | BibTeX | Tags: decentralized, deep learning, federated learning
@inproceedings{kamp2018efficient,
title = {Efficient Decentralized Deep Learning by Dynamic Model Averaging},
author = {Michael Kamp and Linara Adilova and Joachim Sicking and Fabian HĂŒger and Peter Schlicht and Tim Wirtz and Stefan Wrobel},
url = {http://michaelkamp.org/wp-content/uploads/2018/07/commEffDeepLearning_extended.pdf},
year = {2018},
date = {2018-09-14},
urldate = {2018-09-14},
booktitle = {Machine Learning and Knowledge Discovery in Databases},
publisher = {Springer},
abstract = {We propose an efficient protocol for decentralized training of deep neural networks from distributed data sources. The proposed protocol allows to handle different phases of model training equally well and to quickly adapt to concept drifts. This leads to a reduction of communication by an order of magnitude compared to periodically communicating state-of-the-art approaches. Moreover, we derive a communication bound that scales well with the hardness of the serialized learning problem. The reduction in communication comes at almost no cost, as the predictive performance remains virtually unchanged. Indeed, the proposed protocol retains loss bounds of periodically averaging schemes. An extensive empirical evaluation validates major improvement of the trade-off between model performance and communication which could be beneficial for numerous decentralized learning applications, such as autonomous driving, or voice recognition and image classification on mobile phones.},
keywords = {decentralized, deep learning, federated learning},
pubstate = {published},
tppubtype = {inproceedings}
}
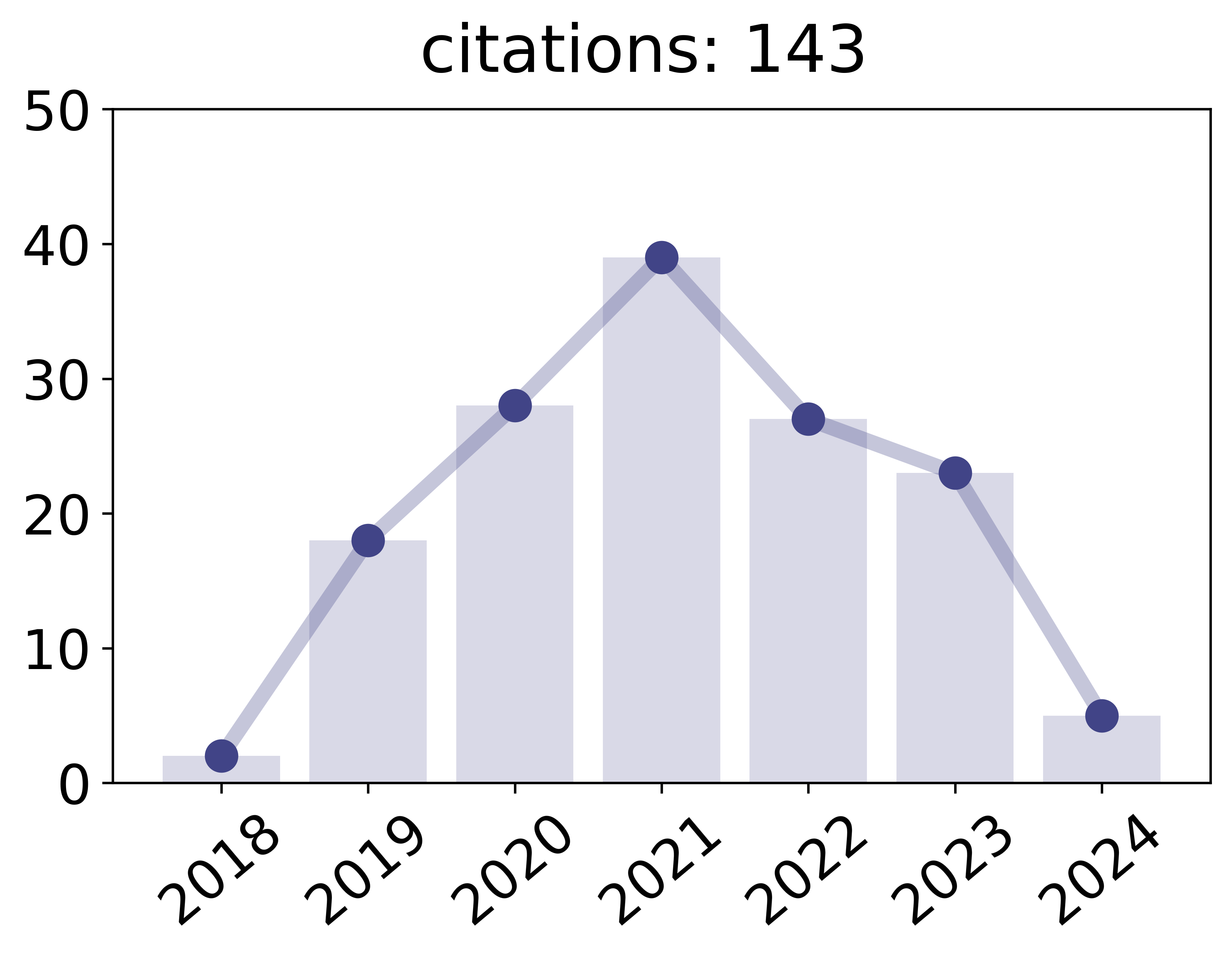
2017
Gunar Ernis, Michael Kamp
Machine Learning fĂŒr die smarte Produktion Journal Article
In: VDMA-Nachrichten, pp. 36-37, 2017.
Links | BibTeX | Tags: industry 4.0, machine learning, smart production
@article{kamp2017machine,
title = {Machine Learning fĂŒr die smarte Produktion},
author = {Gunar Ernis, Michael Kamp},
editor = {Rebecca Pini},
url = {https://sud.vdma.org/documents/15012668/22571546/VDMA-Nachrichten%20Smart%20Data%2011-2017_1513086481204.pdf/c5767569-504e-4f64-8dba-8e7bdd06c18e},
year = {2017},
date = {2017-11-01},
issuetitle = {Smart Data - aus Daten Gold machen},
journal = {VDMA-Nachrichten},
pages = {36-37},
publisher = {Verband Deutscher Maschinen- und Anlagenbau e.V.},
keywords = {industry 4.0, machine learning, smart production},
pubstate = {published},
tppubtype = {article}
}
Flouris, Ioannis; Giatrakos, Nikos; Deligiannakis, Antonios; Garofalakis, Minos; Kamp, Michael; Mock, Michael
Issues in Complex Event Processing: Status and Prospects in the Big Data Era Journal Article
In: Journal of Systems and Software, 2017.
BibTeX | Tags:
@article{flouris2016issues,
title = {Issues in Complex Event Processing: Status and Prospects in the Big Data Era},
author = {Ioannis Flouris and Nikos Giatrakos and Antonios Deligiannakis and Minos Garofalakis and Michael Kamp and Michael Mock},
year = {2017},
date = {2017-01-01},
urldate = {2017-01-01},
journal = {Journal of Systems and Software},
publisher = {Elsevier},
keywords = {},
pubstate = {published},
tppubtype = {article}
}
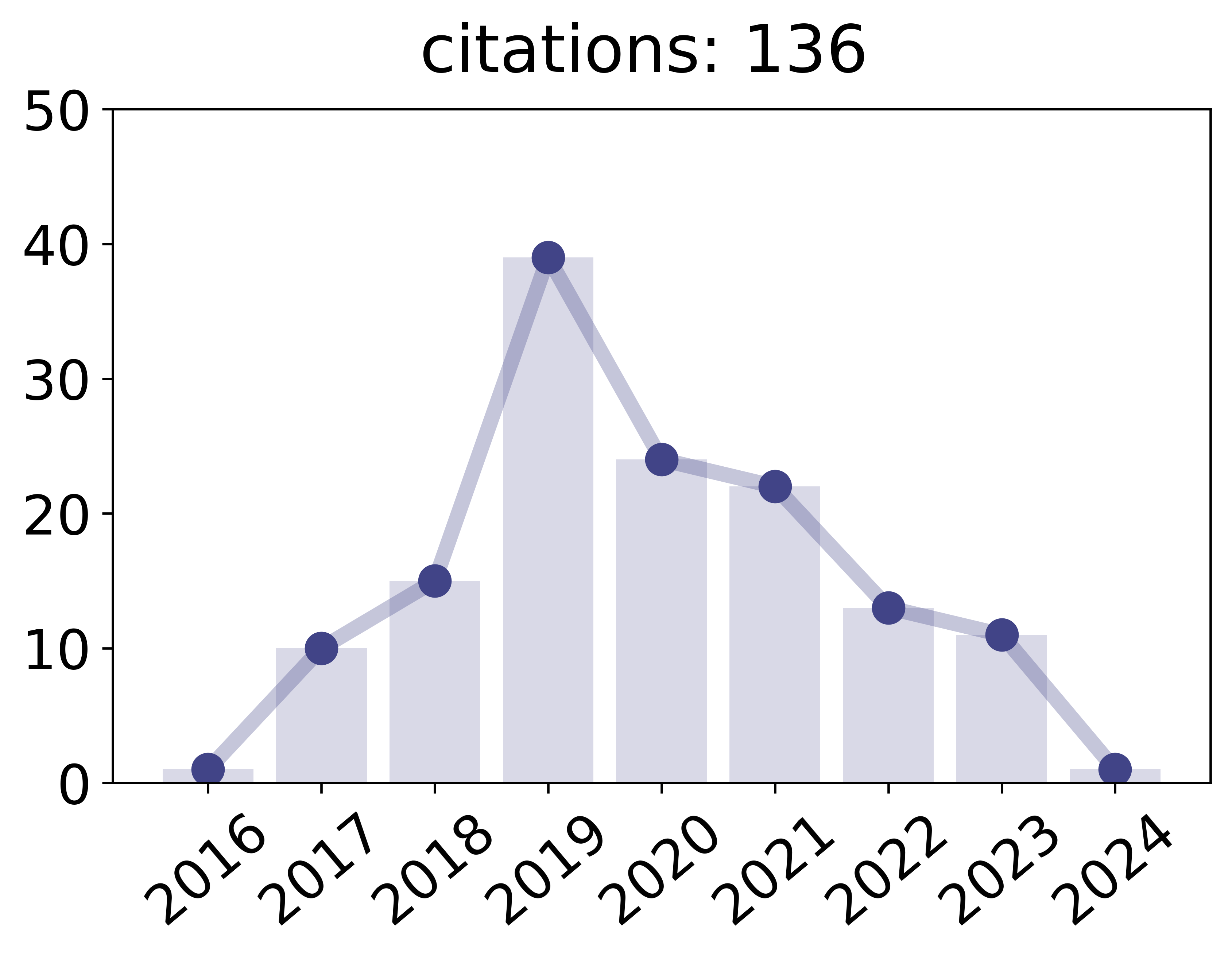
Kamp, Michael; Boley, Mario; Missura, Olana; GĂ€rtner, Thomas
Effective Parallelisation for Machine Learning Proceedings Article
In: Advances in Neural Information Processing Systems, pp. 6480â6491, 2017.
Abstract | Links | BibTeX | Tags: decentralized, distributed, machine learning, parallelization, radon
@inproceedings{kamp2017effective,
title = {Effective Parallelisation for Machine Learning},
author = {Michael Kamp and Mario Boley and Olana Missura and Thomas GĂ€rtner},
url = {http://papers.nips.cc/paper/7226-effective-parallelisation-for-machine-learning.pdf},
year = {2017},
date = {2017-01-01},
urldate = {2017-01-01},
booktitle = {Advances in Neural Information Processing Systems},
pages = {6480--6491},
abstract = {We present a novel parallelisation scheme that simplifies the adaptation of learning algorithms to growing amounts of data as well as growing needs for accurate and confident predictions in critical applications. In contrast to other parallelisation techniques, it can be applied to a broad class of learning algorithms without further mathematical derivations and without writing dedicated code, while at the same time maintaining theoretical performance guarantees. Moreover, our parallelisation scheme is able to reduce the runtime of many learning algorithms to polylogarithmic time on quasi-polynomially many processing units. This is a significant step towards a general answer to an open question on efficient parallelisation of machine learning algorithms in the sense of Nick's Class (NC). The cost of this parallelisation is in the form of a larger sample complexity. Our empirical study confirms the potential of our parallelisation scheme with fixed numbers of processors and instances in realistic application scenarios.},
keywords = {decentralized, distributed, machine learning, parallelization, radon},
pubstate = {published},
tppubtype = {inproceedings}
}
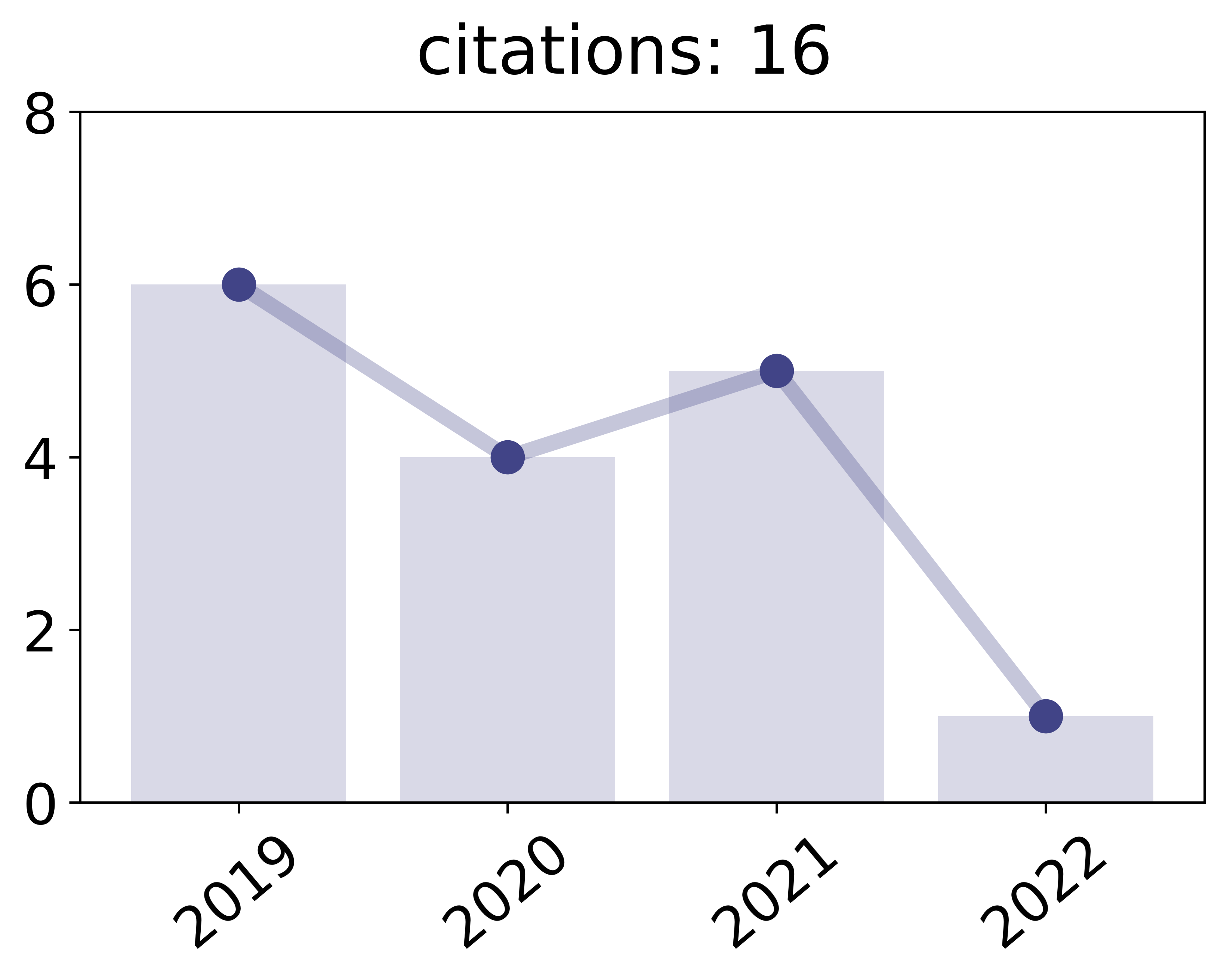
Ullrich, Katrin; Kamp, Michael; GĂ€rtner, Thomas; Vogt, Martin; Wrobel, Stefan
Co-regularised support vector regression Proceedings Article
In: Joint European Conference on Machine Learning and Knowledge Discovery in Databases, pp. 338â354, Springer 2017.
Links | BibTeX | Tags: co-regularization, transfer learning, unsupervised
@inproceedings{ullrich2017co,
title = {Co-regularised support vector regression},
author = {Katrin Ullrich and Michael Kamp and Thomas GĂ€rtner and Martin Vogt and Stefan Wrobel},
url = {http://michaelkamp.org/mk_v1/wp-content/uploads/2018/05/CoRegSVR.pdf},
year = {2017},
date = {2017-01-01},
urldate = {2017-01-01},
booktitle = {Joint European Conference on Machine Learning and Knowledge Discovery in Databases},
pages = {338--354},
organization = {Springer},
keywords = {co-regularization, transfer learning, unsupervised},
pubstate = {published},
tppubtype = {inproceedings}
}
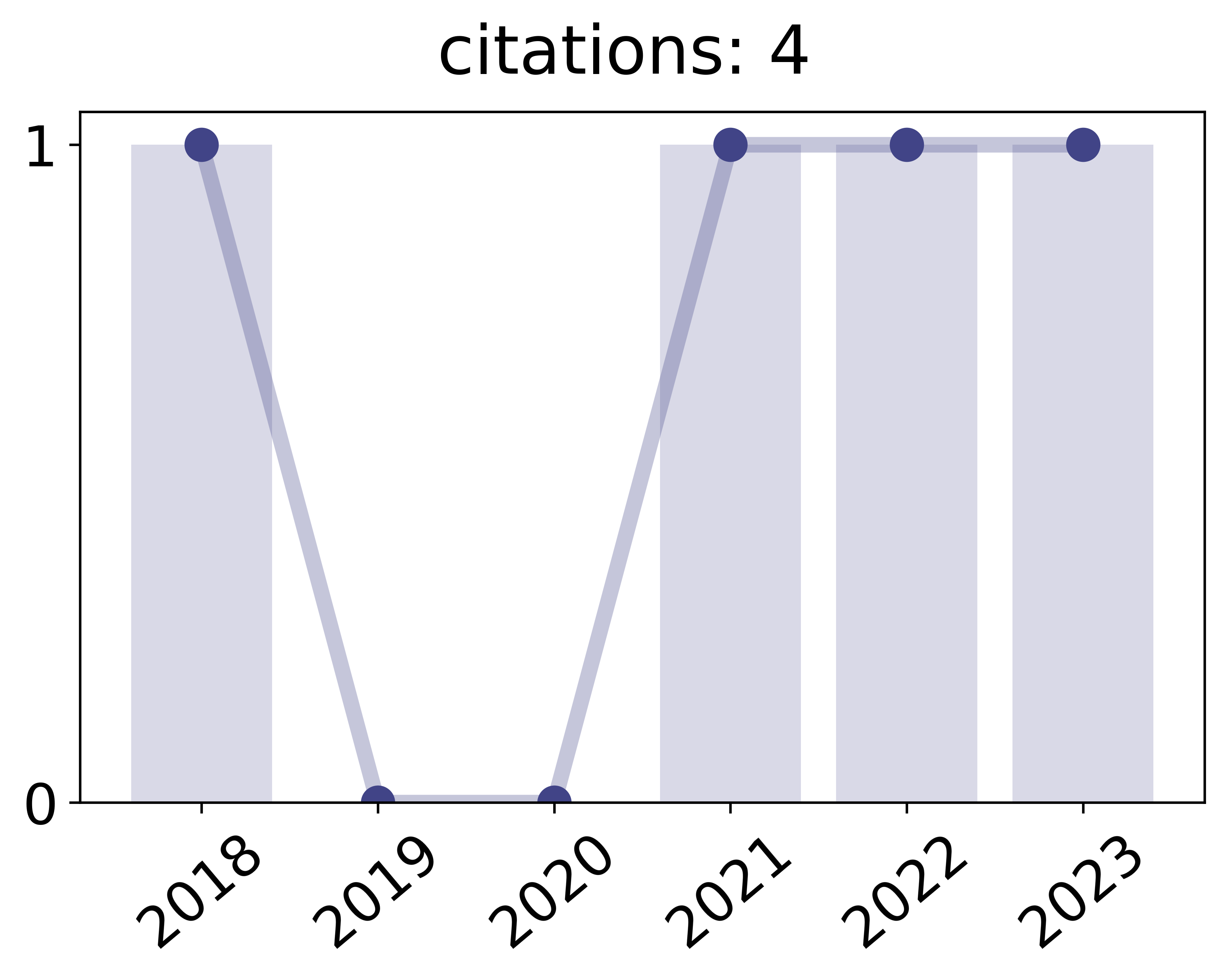
2016
Kamp, Michael; Bothe, Sebastian; Boley, Mario; Mock, Michael
Communication-Efficient Distributed Online Learning with Kernels Proceedings Article
In: Frasconi, Paolo; Landwehr, Niels; Manco, Giuseppe; Vreeken, Jilles (Ed.): Machine Learning and Knowledge Discovery in Databases, pp. 805â819, Springer International Publishing, 2016.
Abstract | Links | BibTeX | Tags: communication-efficient, distributed, dynamic averaging, federated learning, kernel methods, parallelization
@inproceedings{kamp2016communication,
title = {Communication-Efficient Distributed Online Learning with Kernels},
author = {Michael Kamp and Sebastian Bothe and Mario Boley and Michael Mock},
editor = {Paolo Frasconi and Niels Landwehr and Giuseppe Manco and Jilles Vreeken},
url = {http://michaelkamp.org/wp-content/uploads/2020/03/Paper467.pdf},
year = {2016},
date = {2016-09-16},
urldate = {2016-09-16},
booktitle = {Machine Learning and Knowledge Discovery in Databases},
pages = {805--819},
publisher = {Springer International Publishing},
abstract = {We propose an efficient distributed online learning protocol for low-latency real-time services. It extends a previously presented protocol to kernelized online learners that represent their models by a support vector expansion. While such learners often achieve higher predictive performance than their linear counterparts, communicating the support vector expansions becomes inefficient for large numbers of support vectors. The proposed extension allows for a larger class of online learning algorithmsâincluding those alleviating the problem above through model compression. In addition, we characterize the quality of the proposed protocol by introducing a novel criterion that requires the communication to be bounded by the loss suffered.},
keywords = {communication-efficient, distributed, dynamic averaging, federated learning, kernel methods, parallelization},
pubstate = {published},
tppubtype = {inproceedings}
}
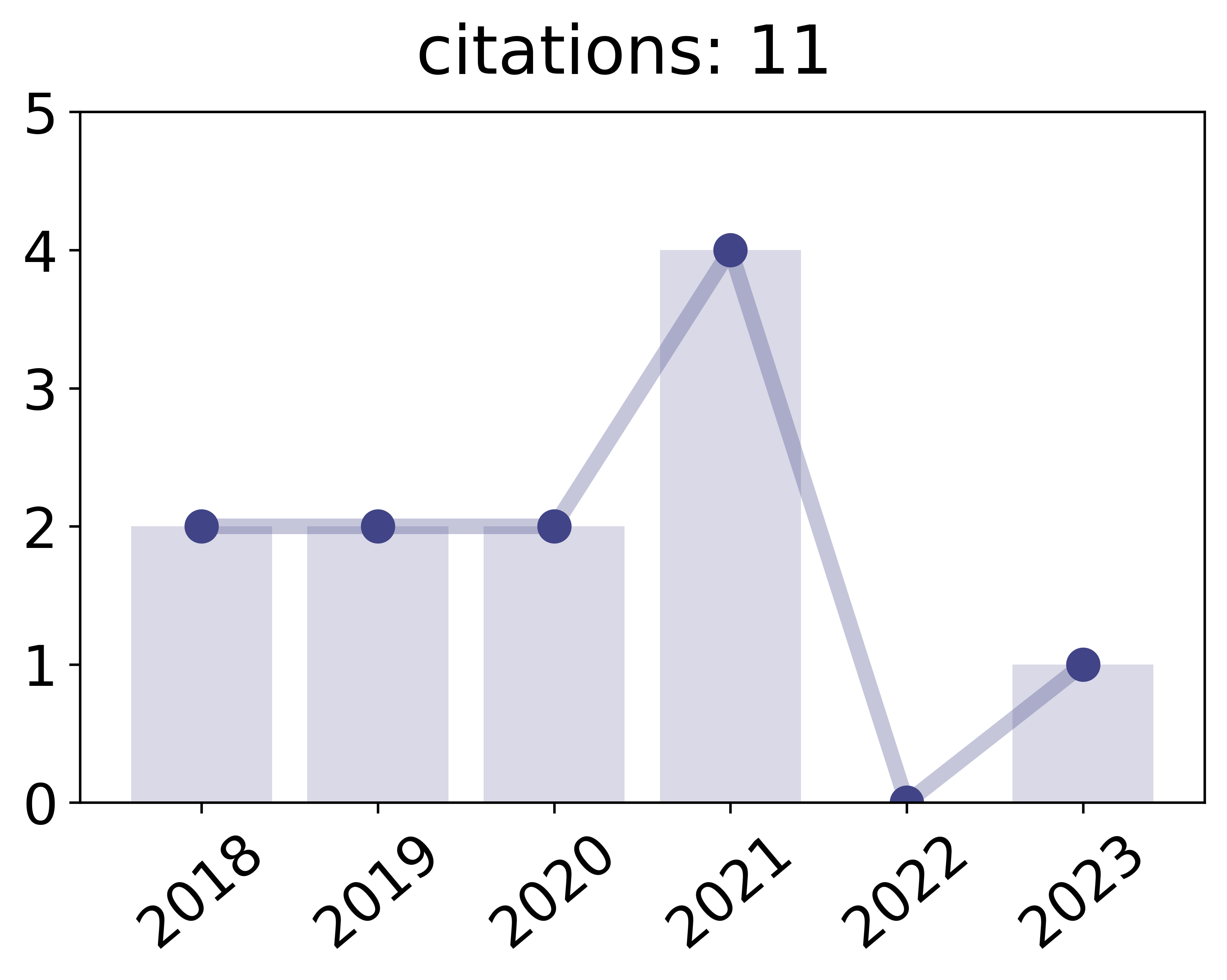
Ullrich, Katrin; Kamp, Michael; GĂ€rtner, Thomas; Vogt, Martin; Wrobel, Stefan
Ligand-based virtual screening with co-regularised support Vector Regression Proceedings Article
In: 2016 IEEE 16th international conference on data mining workshops (ICDMW), pp. 261â268, IEEE 2016.
Abstract | Links | BibTeX | Tags: biology, chemistry, corresponding projections, semi-supervised
@inproceedings{ullrich2016ligand,
title = {Ligand-based virtual screening with co-regularised support Vector Regression},
author = {Katrin Ullrich and Michael Kamp and Thomas GĂ€rtner and Martin Vogt and Stefan Wrobel},
url = {http://michaelkamp.org/wp-content/uploads/2020/03/LigandBasedCoSVR.pdf},
year = {2016},
date = {2016-01-01},
urldate = {2016-01-01},
booktitle = {2016 IEEE 16th international conference on data mining workshops (ICDMW)},
pages = {261--268},
organization = {IEEE},
abstract = {We consider the problem of ligand affinity prediction as a regression task, typically with few labelled examples, many unlabelled instances, and multiple views on the data. In chemoinformatics, the prediction of binding affinities for protein ligands is an important but also challenging task. As protein-ligand bonds trigger biochemical reactions, their characterisation is a crucial step in the process of drug discovery and design. However, the practical determination of ligand affinities is very expensive, whereas unlabelled compounds are available in abundance. Additionally, many different vectorial representations for compounds (molecular fingerprints) exist that cover different sets of features. To this task we propose to apply a co-regularisation approach, which extracts information from unlabelled examples by ensuring that individual models trained on different fingerprints make similar predictions. We extend support vector regression similarly to the existing co-regularised least squares regression (CoRLSR) and obtain a co-regularised support vector regression (CoSVR). We empirically evaluate the performance of CoSVR on various protein-ligand datasets. We show that CoSVR outperforms CoRLSR as well as existing state-of-the-art approaches that do not take unlabelled molecules into account. Additionally, we provide a theoretical bound on the Rademacher complexity for CoSVR.},
keywords = {biology, chemistry, corresponding projections, semi-supervised},
pubstate = {published},
tppubtype = {inproceedings}
}
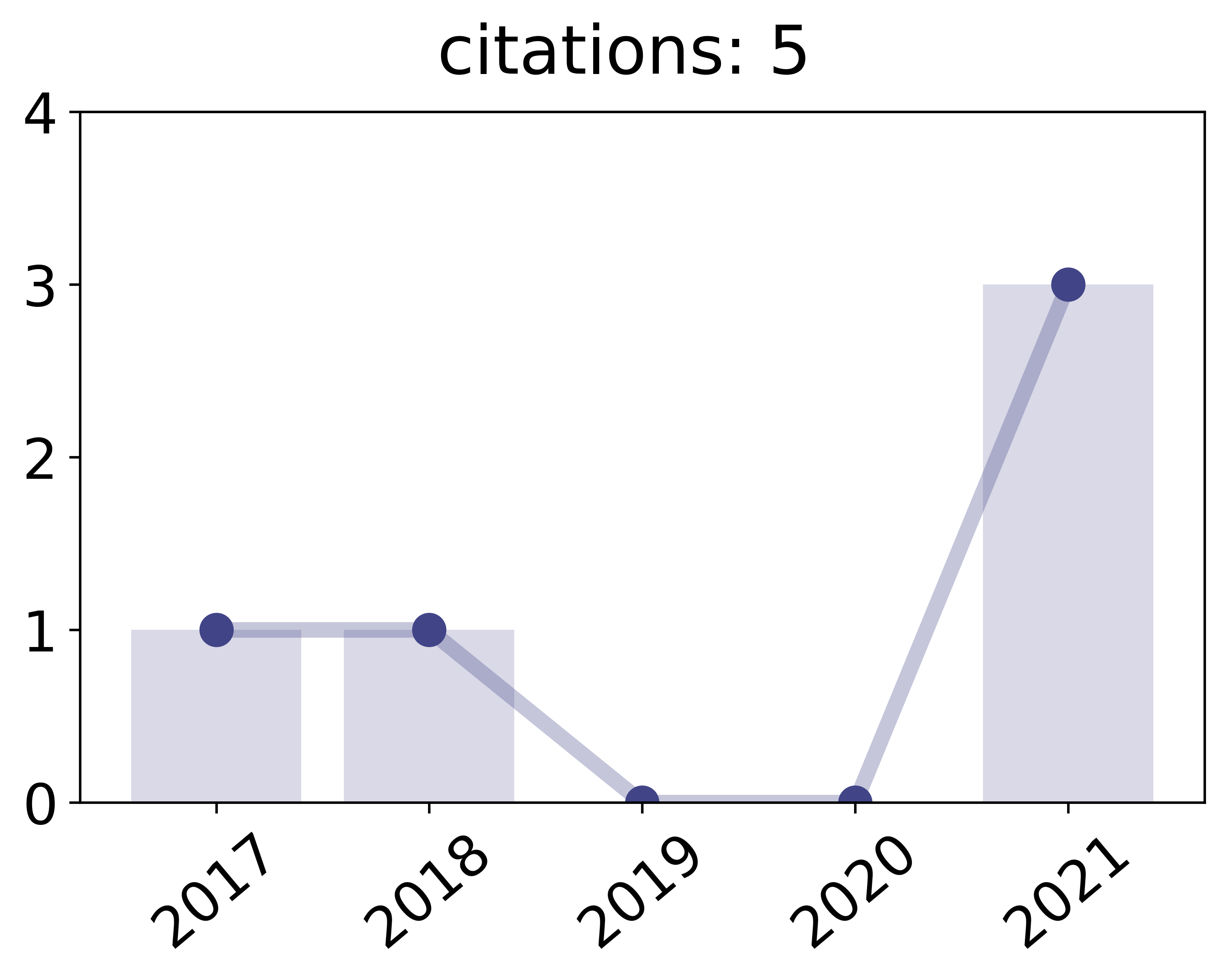
2015
Kamp, Michael; Boley, Mario; GĂ€rtner, Thomas
Parallelizing Randomized Convex Optimization Workshop
Proceedings of the 8th NIPS Workshop on Optimization for Machine Learning, 2015.
@workshop{kamp2015parallelizing,
title = {Parallelizing Randomized Convex Optimization},
author = {Michael Kamp and Mario Boley and Thomas GĂ€rtner},
url = {http://www.opt-ml.org/papers/OPT2015_paper_23.pdf},
year = {2015},
date = {2015-01-01},
urldate = {2015-01-01},
booktitle = {Proceedings of the 8th NIPS Workshop on Optimization for Machine Learning},
keywords = {},
pubstate = {published},
tppubtype = {workshop}
}
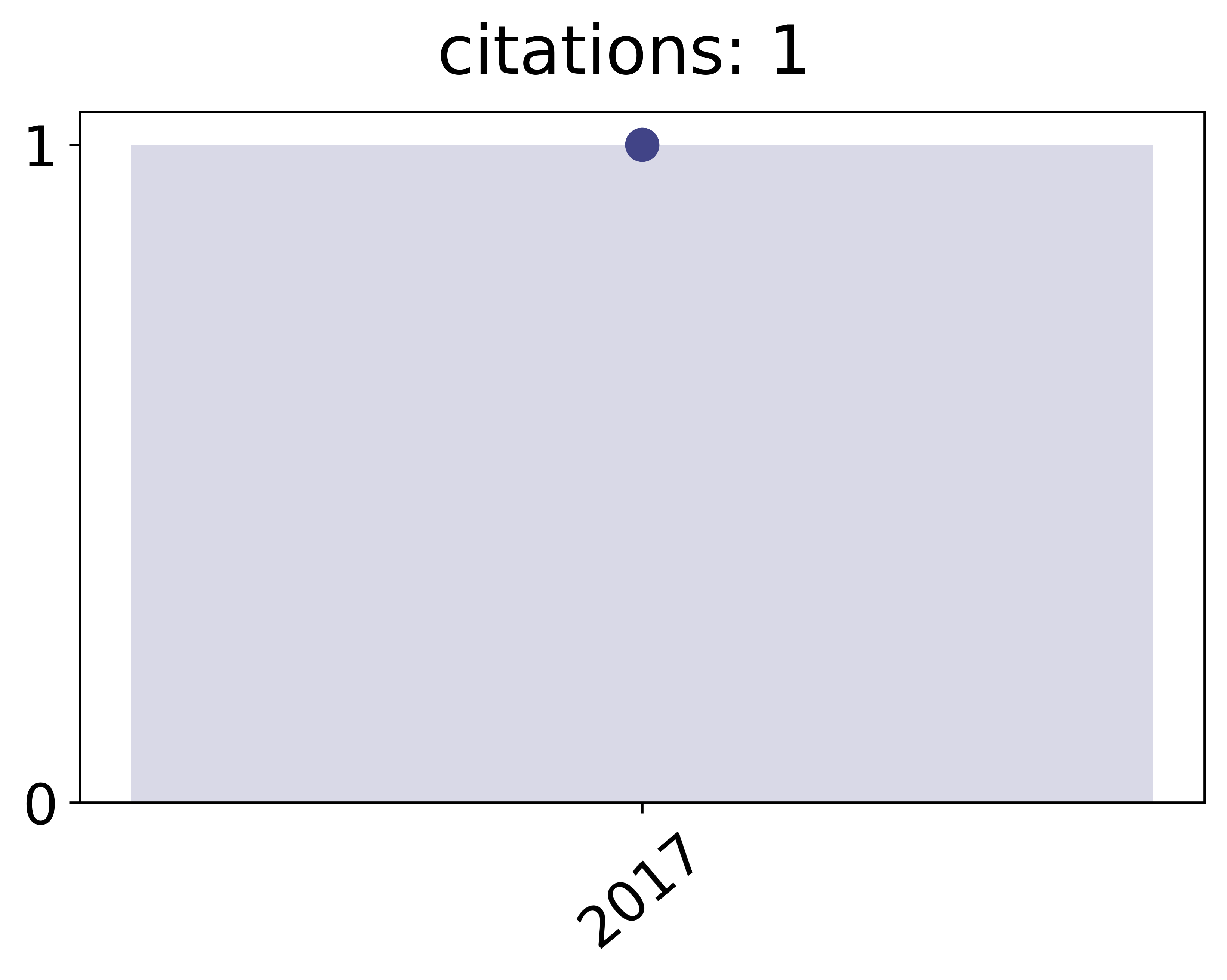
2014
Kamp, Michael; Boley, Mario; GĂ€rtner, Thomas
Beating Human Analysts in Nowcasting Corporate Earnings by using Publicly Available Stock Price and Correlation Features Proceedings Article
In: Proceedings of the SIAM International Conference on Data Mining, pp. 641â649, SIAM 2014.
@inproceedings{michael2014beating,
title = {Beating Human Analysts in Nowcasting Corporate Earnings by using Publicly Available Stock Price and Correlation Features},
author = {Michael Kamp and Mario Boley and Thomas GĂ€rtner},
url = {http://www.ferari-project.eu/wp-content/uploads/2014/12/earningsPrediction.pdf},
year = {2014},
date = {2014-01-01},
urldate = {2014-01-01},
booktitle = {Proceedings of the SIAM International Conference on Data Mining},
volume = {72},
pages = {641--649},
organization = {SIAM},
keywords = {},
pubstate = {published},
tppubtype = {inproceedings}
}
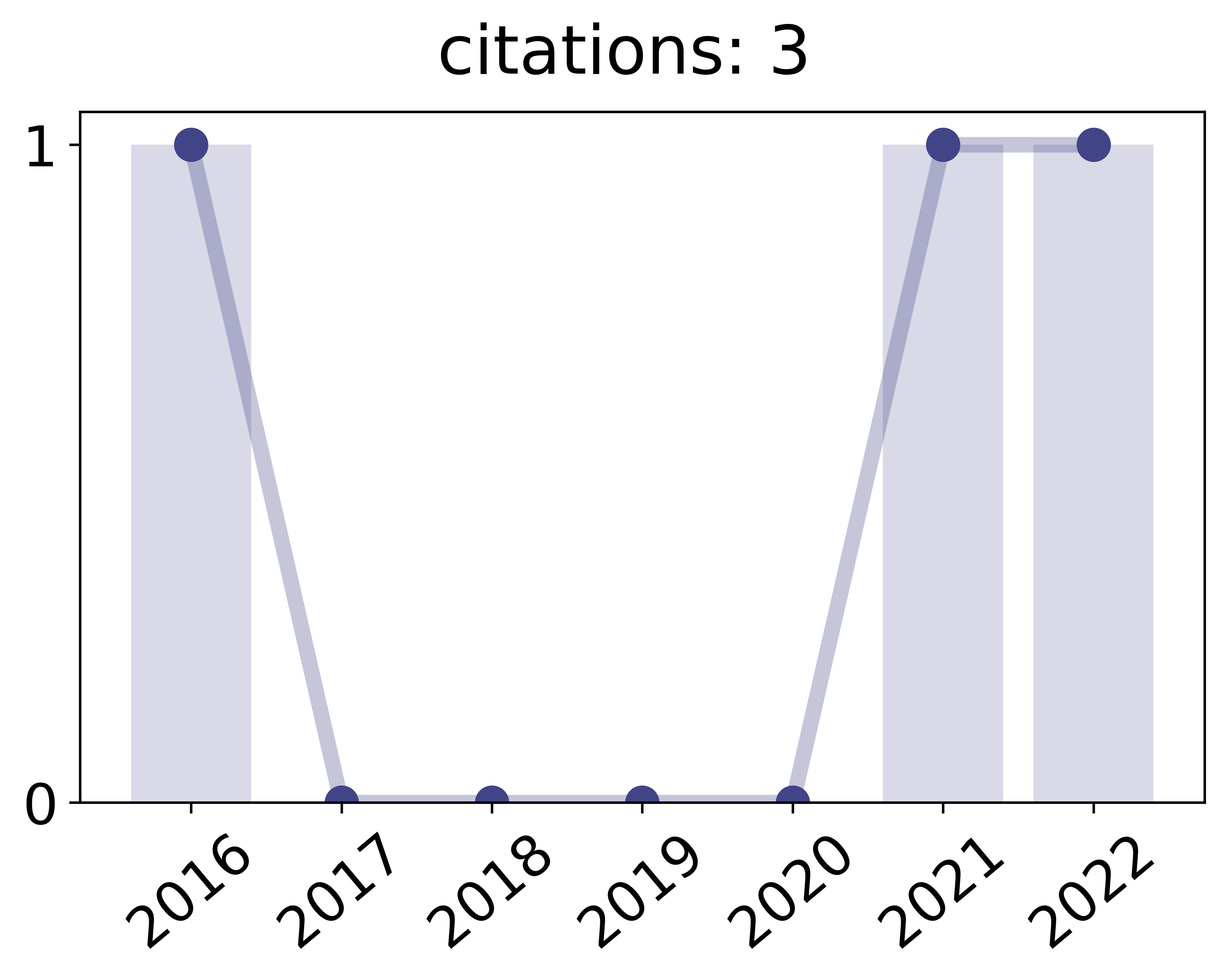
Kamp, Michael; Boley, Mario; Keren, Daniel; Schuster, Assaf; Sharfman, Izchak
Communication-Efficient Distributed Online Prediction by Dynamic Model Synchronization Proceedings Article
In: European Conference on Machine Learning and Principles and Practice of Knowledge Discovery (ECMLPKDD), Springer 2014.
BibTeX | Tags:
@inproceedings{kamp2014communication,
title = {Communication-Efficient Distributed Online Prediction by Dynamic Model Synchronization},
author = {Michael Kamp and Mario Boley and Daniel Keren and Assaf Schuster and Izchak Sharfman},
year = {2014},
date = {2014-01-01},
urldate = {2014-01-01},
booktitle = {European Conference on Machine Learning and Principles and Practice of Knowledge Discovery (ECMLPKDD)},
organization = {Springer},
keywords = {},
pubstate = {published},
tppubtype = {inproceedings}
}
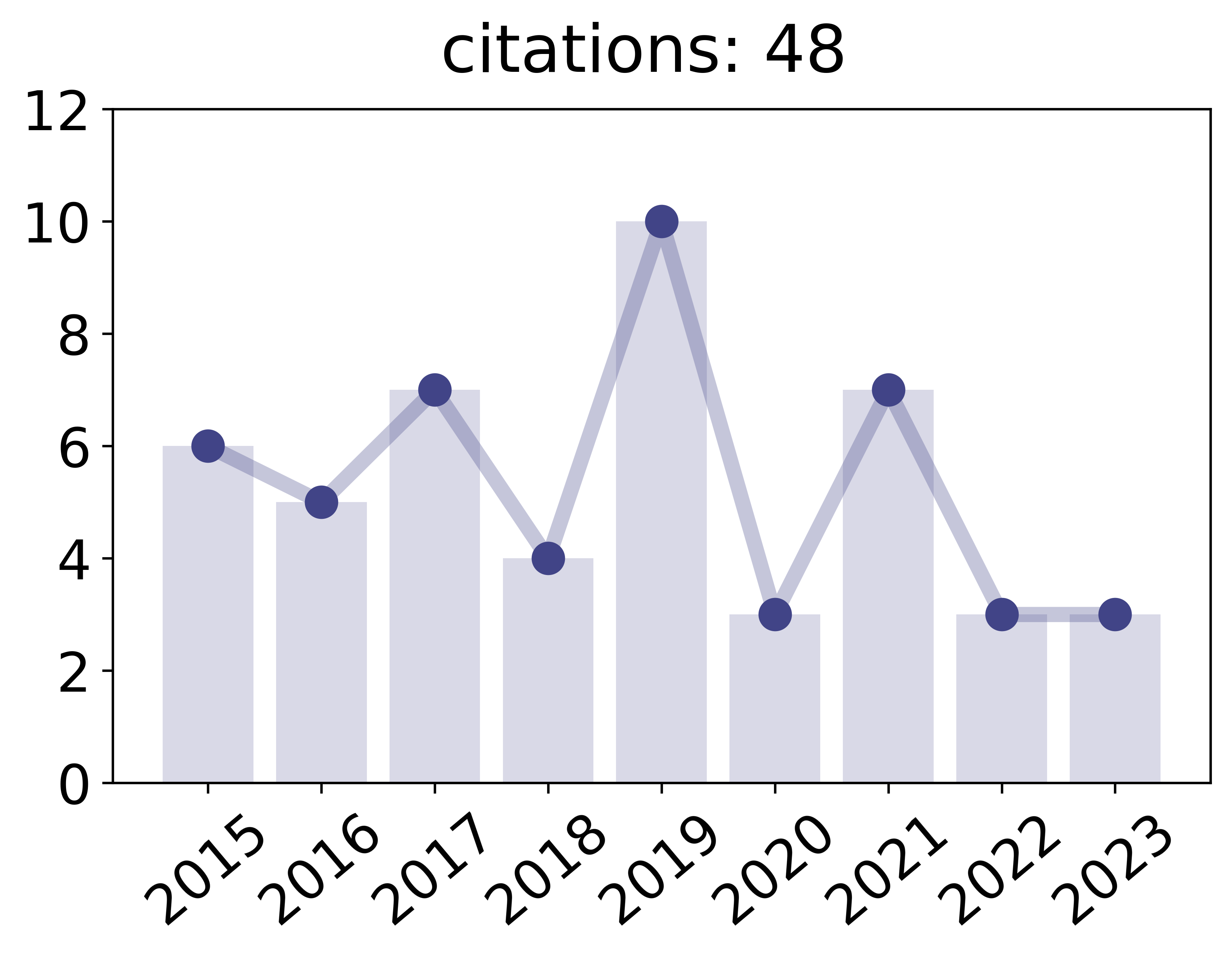
Kamp, Michael; Boley, Mario; Mock, Michael; Keren, Daniel; Schuster, Assaf; Sharfman, Izchak
Adaptive Communication Bounds for Distributed Online Learning Workshop
Proceedings of the 7th NIPS Workshop on Optimization for Machine Learning, 2014.
@workshop{kamp2014adaptive,
title = {Adaptive Communication Bounds for Distributed Online Learning},
author = {Michael Kamp and Mario Boley and Michael Mock and Daniel Keren and Assaf Schuster and Izchak Sharfman},
url = {https://arxiv.org/abs/1911.12896},
year = {2014},
date = {2014-01-01},
urldate = {2014-01-01},
booktitle = {Proceedings of the 7th NIPS Workshop on Optimization for Machine Learning},
keywords = {},
pubstate = {published},
tppubtype = {workshop}
}
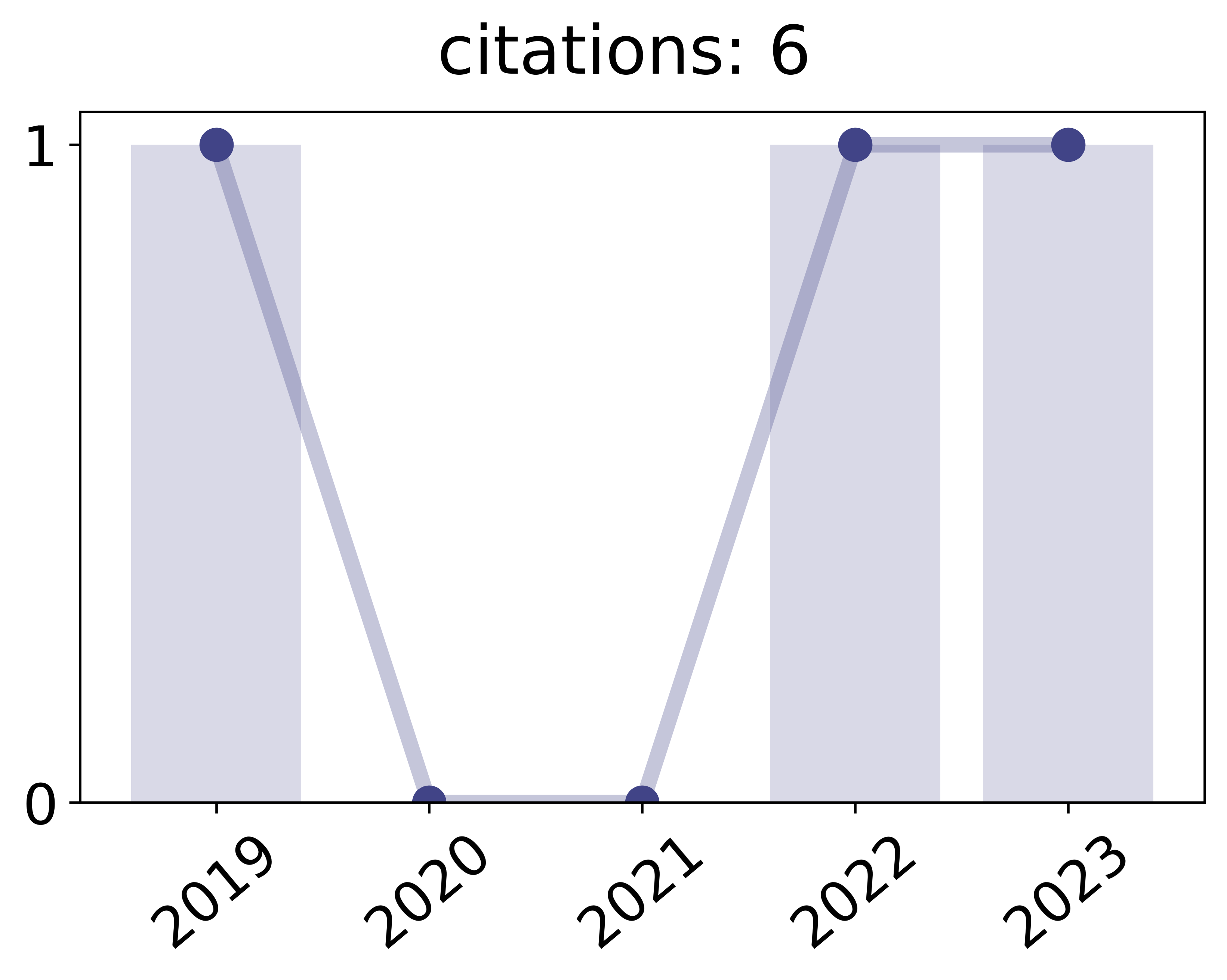
2013
Kamp, Michael; Kopp, Christine; Mock, Michael; Boley, Mario; May, Michael
Privacy-preserving mobility monitoring using sketches of stationary sensor readings Proceedings Article
In: Joint European Conference on Machine Learning and Knowledge Discovery in Databases, pp. 370â386, Springer 2013.
BibTeX | Tags:
@inproceedings{kamp2013privacy,
title = {Privacy-preserving mobility monitoring using sketches of stationary sensor readings},
author = {Michael Kamp and Christine Kopp and Michael Mock and Mario Boley and Michael May},
year = {2013},
date = {2013-01-01},
urldate = {2013-01-01},
booktitle = {Joint European Conference on Machine Learning and Knowledge Discovery in Databases},
pages = {370--386},
organization = {Springer},
keywords = {},
pubstate = {published},
tppubtype = {inproceedings}
}
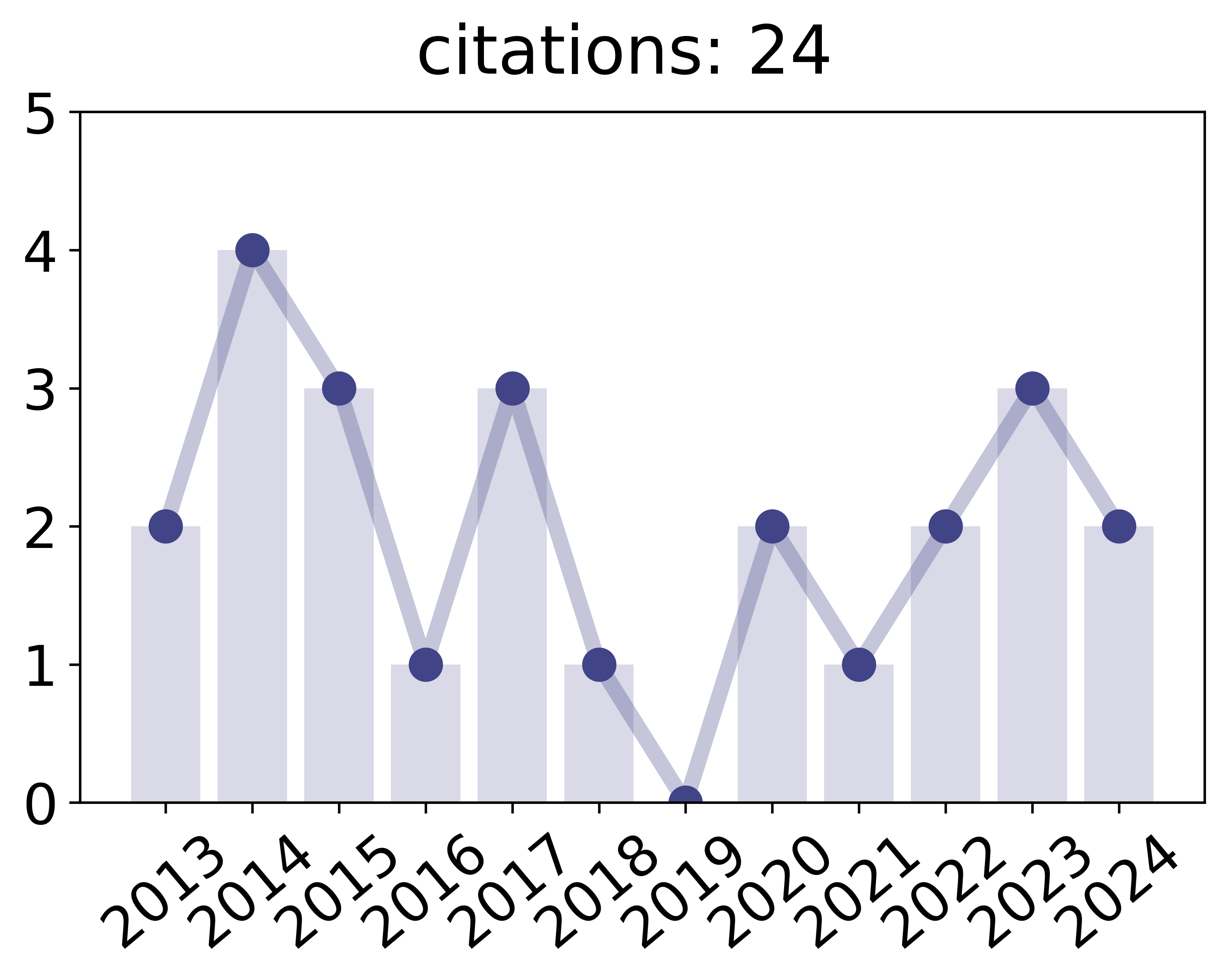
Kamp, Michael; Boley, Mario; GĂ€rtner, Thomas
2013 IEEE 13th International Conference on Data Mining Workshops, IEEE 2013.
BibTeX | Tags:
@workshop{kamp2013beating,
title = {Beating Human Analysts in Nowcasting Corporate Earnings by Using Publicly Available Stock Price and Correlation Features},
author = {Michael Kamp and Mario Boley and Thomas GĂ€rtner},
year = {2013},
date = {2013-01-01},
urldate = {2013-01-01},
booktitle = {2013 IEEE 13th International Conference on Data Mining Workshops},
pages = {384--390},
organization = {IEEE},
keywords = {},
pubstate = {published},
tppubtype = {workshop}
}
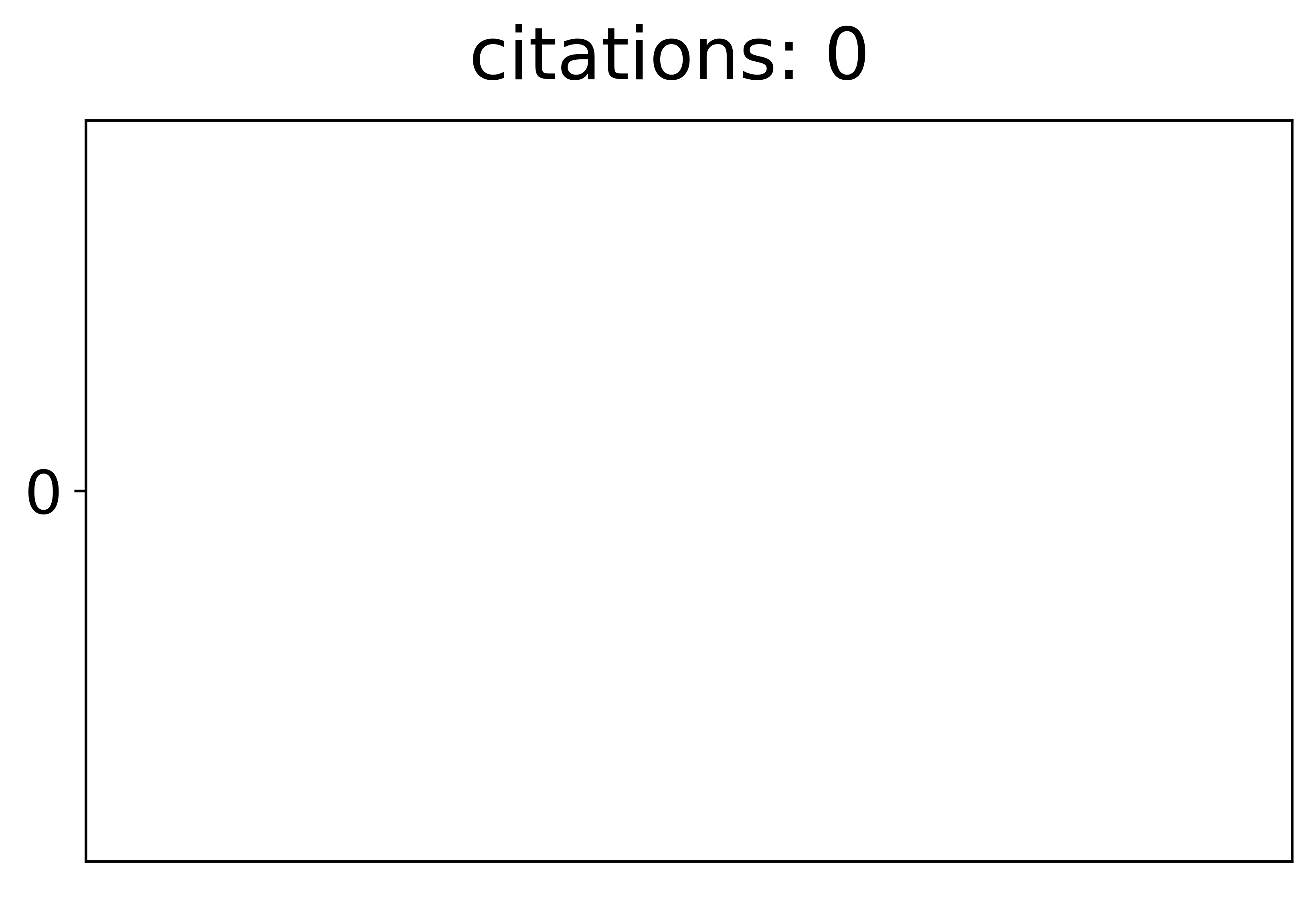
Boley, Mario; Kamp, Michael; Keren, Daniel; Schuster, Assaf; Sharfman, Izchak
Communication-Efficient Distributed Online Prediction using Dynamic Model Synchronizations. Workshop
First Internation Workshop on Big Dynamic Distributed Data (BD3) at the Internation Conference on Very Large Data Bases (VLDB), 2013.
BibTeX | Tags:
@workshop{boley2013communication,
title = {Communication-Efficient Distributed Online Prediction using Dynamic Model Synchronizations.},
author = {Mario Boley and Michael Kamp and Daniel Keren and Assaf Schuster and Izchak Sharfman},
year = {2013},
date = {2013-01-01},
urldate = {2013-01-01},
booktitle = {First Internation Workshop on Big Dynamic Distributed Data (BD3) at the Internation Conference on Very Large Data Bases (VLDB)},
pages = {13--18},
keywords = {},
pubstate = {published},
tppubtype = {workshop}
}
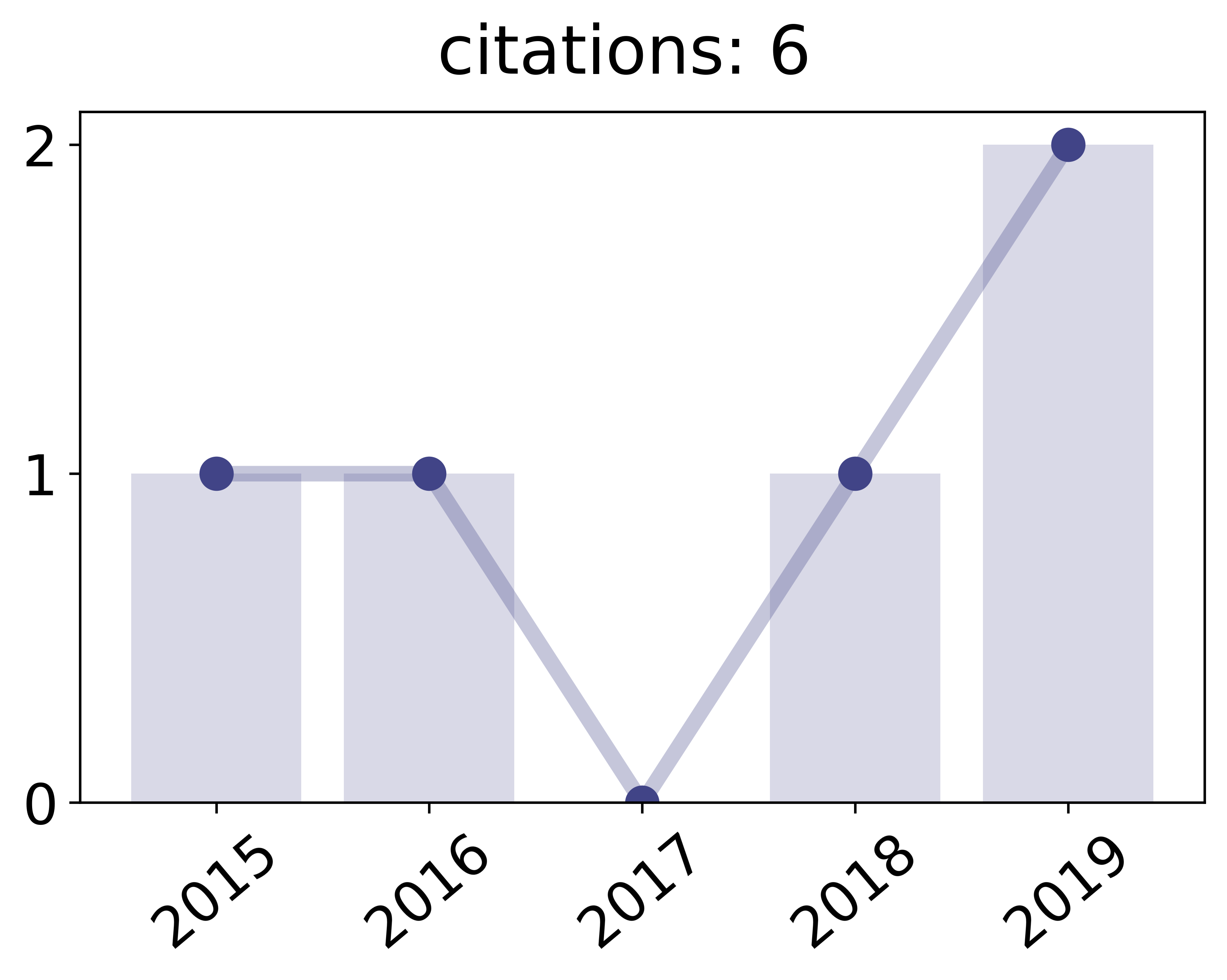
Kamp, Michael; Manea, Andrei
STONES: Stochastic Technique for Generating Songs Workshop
Proceedings of the NIPS Workshop on Constructive Machine Learning (CML), 2013.
BibTeX | Tags:
@workshop{kamp2013stones,
title = {STONES: Stochastic Technique for Generating Songs},
author = {Michael Kamp and Andrei Manea},
year = {2013},
date = {2013-01-01},
urldate = {2013-01-01},
booktitle = {Proceedings of the NIPS Workshop on Constructive Machine Learning (CML)},
keywords = {},
pubstate = {published},
tppubtype = {workshop}
}
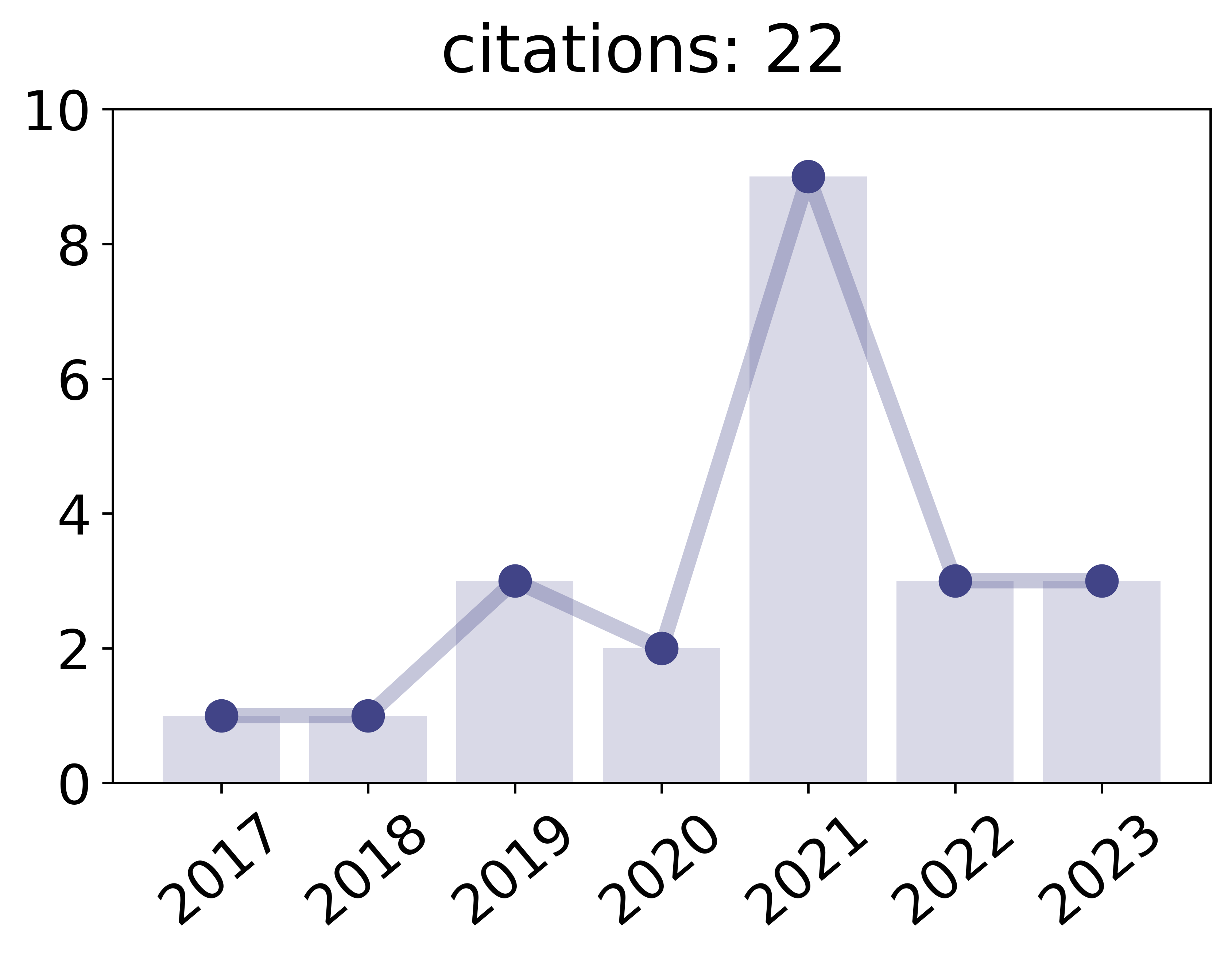